Recruiting the right talent for statistics roles can be challenging, especially with varying levels of expertise required. Understanding the right questions to ask in an interview is essential to identifying the best candidates and ensuring team success.
In this blog post, we'll provide you with a comprehensive list of statistics interview questions categorized by skill level and specific statistical concepts. From junior analysts to senior experts, and topics like probability theory and regression analysis, we've got you covered.
By using these questions, you can better evaluate candidates' statistical knowledge and problem-solving abilities. Additionally, consider utilizing our statistics online test to streamline your pre-interview assessment process and ensure you’re interviewing only the best candidates.
Table of contents
10 basic Statistics interview questions and answers to assess candidates
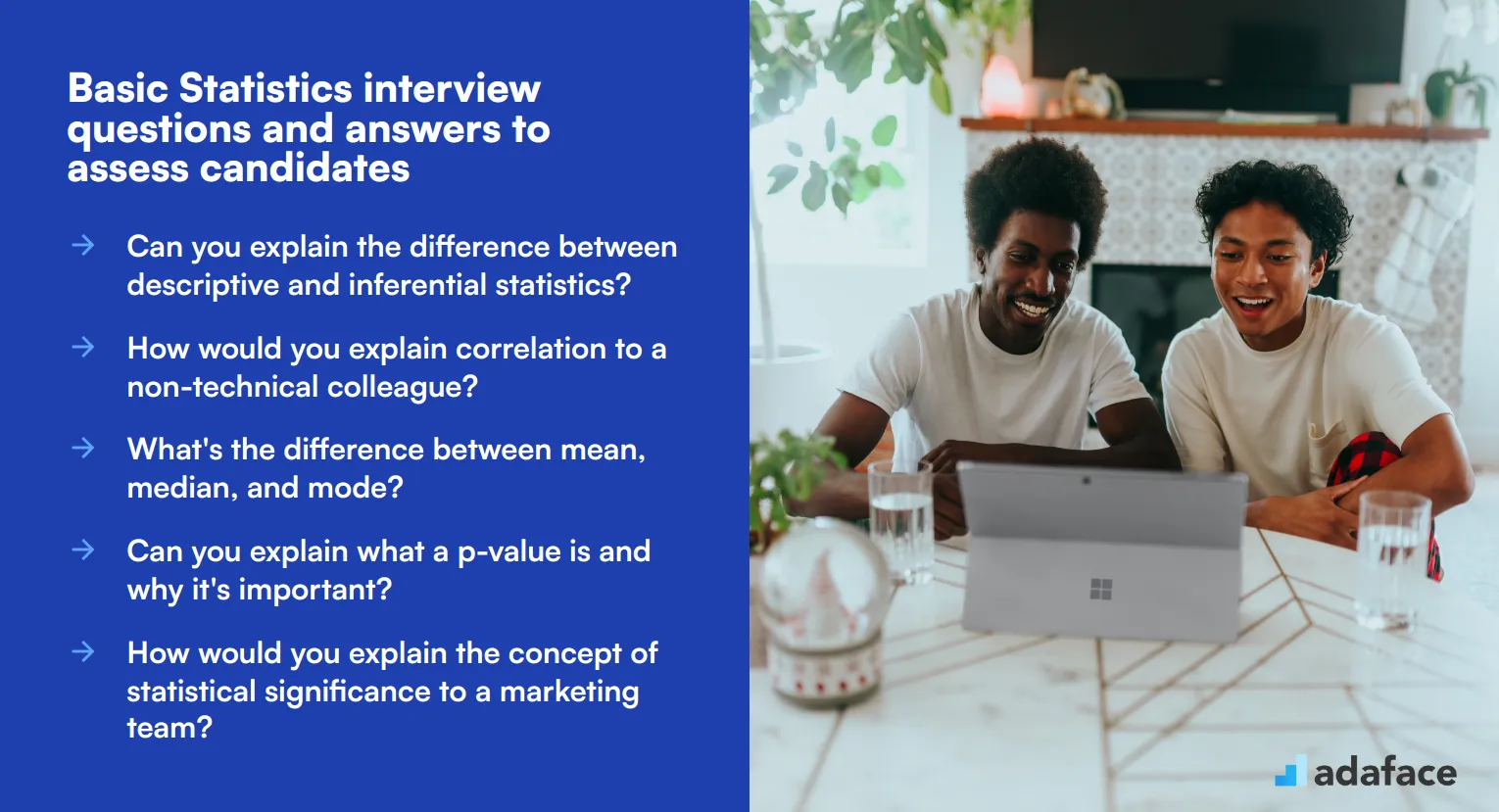
Ready to assess your statistics candidates? These 10 basic questions will help you gauge their understanding of fundamental concepts and their ability to apply statistical thinking in real-world scenarios. Use this list to spark meaningful discussions and uncover candidates' potential to contribute to your data-driven decision-making processes.
1. Can you explain the difference between descriptive and inferential statistics?
Descriptive statistics summarize and describe the main features of a dataset. This includes measures of central tendency (mean, median, mode) and measures of variability (range, standard deviation). Descriptive statistics help us understand the basic characteristics of our data.
Inferential statistics, on the other hand, use sample data to make predictions or inferences about a larger population. This involves hypothesis testing, estimation, and drawing conclusions that extend beyond the immediate data alone.
Look for candidates who can clearly differentiate between these two types and provide examples of when each would be used in real-world scenarios. Strong candidates might also mention the importance of data quality in both descriptive and inferential statistics.
2. How would you explain correlation to a non-technical colleague?
A good answer might sound like this: 'Correlation is a way to measure how two things change together. Imagine you're watching ice cream sales and temperature over a summer. If ice cream sales go up when it's hotter, and down when it's cooler, we'd say there's a positive correlation between temperature and ice cream sales.'
The candidate should emphasize that correlation doesn't imply causation. They might add, 'Just because two things are correlated doesn't mean one causes the other. For example, ice cream sales and sunburn cases might be correlated, but eating ice cream doesn't cause sunburns!'
Look for candidates who can explain complex concepts in simple terms and who understand the limitations of statistical measures. Strong candidates might also mention different types of correlation (positive, negative, no correlation) and how correlation strength is measured.
3. What's the difference between mean, median, and mode?
The mean is the average of all numbers in a dataset, calculated by summing all values and dividing by the count of values. The median is the middle value when the dataset is ordered from lowest to highest. If there's an even number of values, it's the average of the two middle numbers. The mode is the value that appears most frequently in the dataset.
A good answer should include examples: 'For the dataset [1, 2, 2, 3, 4, 5], the mean is 2.83, the median is 2.5, and the mode is 2.'
Look for candidates who can explain when each measure is most appropriate. For instance, they might mention that median is often used for income data because it's less affected by extreme values than the mean. This demonstrates a practical understanding of data analysis concepts.
4. Can you explain what a p-value is and why it's important?
A p-value is a probability that measures the evidence against a null hypothesis. It represents the likelihood of obtaining results at least as extreme as the observed results, assuming that the null hypothesis is true. Typically, a p-value less than 0.05 is considered statistically significant, leading to the rejection of the null hypothesis.
For example, if we're testing whether a new drug is effective and we get a p-value of 0.03, it means there's only a 3% chance we'd see results this extreme if the drug had no effect. This suggests the drug likely does have an effect.
Look for candidates who can explain this concept clearly and who understand its limitations. Strong candidates might mention that p-values don't measure the size or importance of an effect, only its statistical significance. They might also discuss the ongoing debates about p-value thresholds in scientific research.
5. How would you explain the concept of statistical significance to a marketing team?
A good explanation might go like this: 'Statistical significance helps us determine if a result is likely due to chance or if it represents a real effect. Imagine we're testing two versions of an ad. Version A got a 5% click-through rate, and version B got a 5.5% rate. Statistical significance tells us whether this difference is big enough to be meaningful, or if it might just be random variation.'
The candidate should mention that statistical significance is typically set at a 95% confidence level (p < 0.05). They might add, 'This means we're 95% confident that the difference we're seeing is real and not just due to chance.'
Look for candidates who can relate statistical concepts to business scenarios. Strong candidates might discuss the balance between statistical significance and practical significance, noting that a statistically significant result isn't always practically important for business decisions.
6. What is the difference between population and sample in statistics?
A population in statistics refers to the entire group of individuals or items that we want to draw conclusions about. For example, if we're studying voting preferences, the population might be all registered voters in a country. A sample, on the other hand, is a subset of the population that we actually collect data from and analyze.
Candidates should explain that we often use samples because studying entire populations can be impractical or impossible. They might say, 'We can't ask every voter in the country about their preferences, so we survey a representative sample instead.'
Look for candidates who understand the importance of random sampling and can explain concepts like sampling error and bias. Strong candidates might discuss different sampling methods and their applications in various data science scenarios.
7. Can you explain what a confidence interval is?
A confidence interval is a range of values that's likely to contain the true population parameter with a certain level of confidence. For example, if we say 'we're 95% confident that the average height of adult males is between 68 and 72 inches,' we're providing a 95% confidence interval for the population mean height.
Candidates should explain that wider intervals indicate less precision but more certainty, while narrower intervals indicate more precision but less certainty. They might add, 'If we want to be more confident, say 99% instead of 95%, our interval would be wider.'
Look for candidates who can explain how confidence intervals are used in real-world decision-making. Strong candidates might discuss factors that affect the width of confidence intervals, such as sample size and population variability.
8. How would you explain the concept of regression to the marketing department?
A good explanation might go: 'Regression is a statistical method that helps us understand how different factors influence an outcome we're interested in. For example, we might use regression to see how factors like advertising spend, social media engagement, and seasonality affect our sales.'
The candidate should emphasize that regression can help predict outcomes and identify which factors have the strongest influence. They might add, 'If our regression model shows that social media engagement has a strong positive relationship with sales, we might decide to invest more in our social media strategy.'
Look for candidates who can explain complex statistical concepts in business terms. Strong candidates might discuss different types of regression (like linear or logistic) and when each is appropriate. They should also be able to explain limitations, such as the fact that regression shows correlation, not causation.
9. What is the difference between Type I and Type II errors?
Type I error, also known as a false positive, occurs when we reject a null hypothesis that is actually true. Type II error, or false negative, occurs when we fail to reject a null hypothesis that is actually false. A candidate might explain it like this: 'If we're testing a new drug, a Type I error would be concluding the drug works when it actually doesn't. A Type II error would be concluding the drug doesn't work when it actually does.'
Candidates should mention that there's always a trade-off between these two types of errors. Reducing the chance of one type of error typically increases the chance of the other.
Look for candidates who can discuss the implications of each type of error in real-world scenarios. Strong candidates might mention how the choice of significance level affects the likelihood of Type I errors, or how increasing sample size can help reduce Type II errors.
10. How would you explain the importance of data visualization in statistics?
Data visualization is crucial in statistics because it helps us understand and communicate complex data patterns quickly and effectively. A good answer might include: 'Visualizations can reveal trends, outliers, and relationships in data that might not be obvious from looking at raw numbers or summary statistics.'
Candidates should be able to discuss various types of visualizations and when to use them. For example, 'We might use a scatter plot to show the relationship between two variables, a bar chart to compare categories, or a line graph to show changes over time.'
Look for candidates who understand that effective data visualization is about more than just making things look pretty. Strong candidates might discuss principles of good data visualization, such as choosing appropriate scales, using color effectively, and avoiding chart junk. They might also mention tools commonly used for data visualization in data analysis roles.
20 Statistics interview questions to ask junior analysts
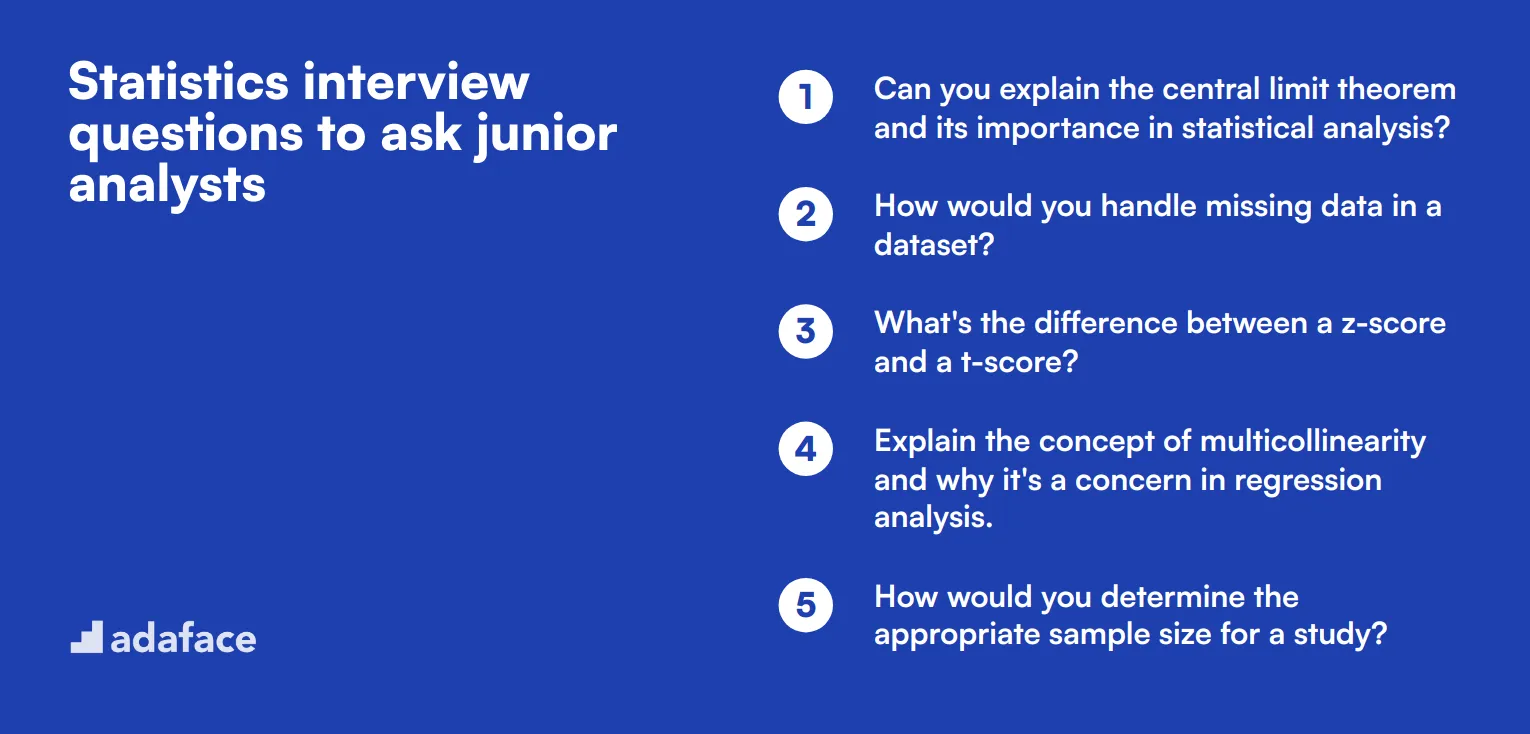
To assess the statistical knowledge of junior analysts, use these 20 interview questions. They cover fundamental concepts and practical applications, helping you gauge candidates' understanding and problem-solving abilities in real-world scenarios.
- Can you explain the central limit theorem and its importance in statistical analysis?
- How would you handle missing data in a dataset?
- What's the difference between a z-score and a t-score?
- Explain the concept of multicollinearity and why it's a concern in regression analysis.
- How would you determine the appropriate sample size for a study?
- What is the difference between parametric and non-parametric tests?
- Explain the concept of Simpson's paradox with a simple example.
- How would you detect and handle outliers in a dataset?
- What is the difference between a histogram and a bar chart?
- Explain the concept of statistical power and its importance in hypothesis testing.
- How would you choose between a chi-square test and a t-test?
- What is the purpose of data normalization, and when would you use it?
- Explain the difference between correlation and causation with a real-world example.
- How would you interpret a box plot?
- What is the purpose of bootstrapping in statistics?
- Explain the concept of heteroscedasticity and its impact on regression analysis.
- How would you handle skewed data in your analysis?
- What is the difference between a discrete and continuous variable?
- Explain the concept of degrees of freedom in statistical analysis.
- How would you approach A/B testing for a website redesign?
10 intermediate Statistics interview questions and answers to ask mid-tier analysts
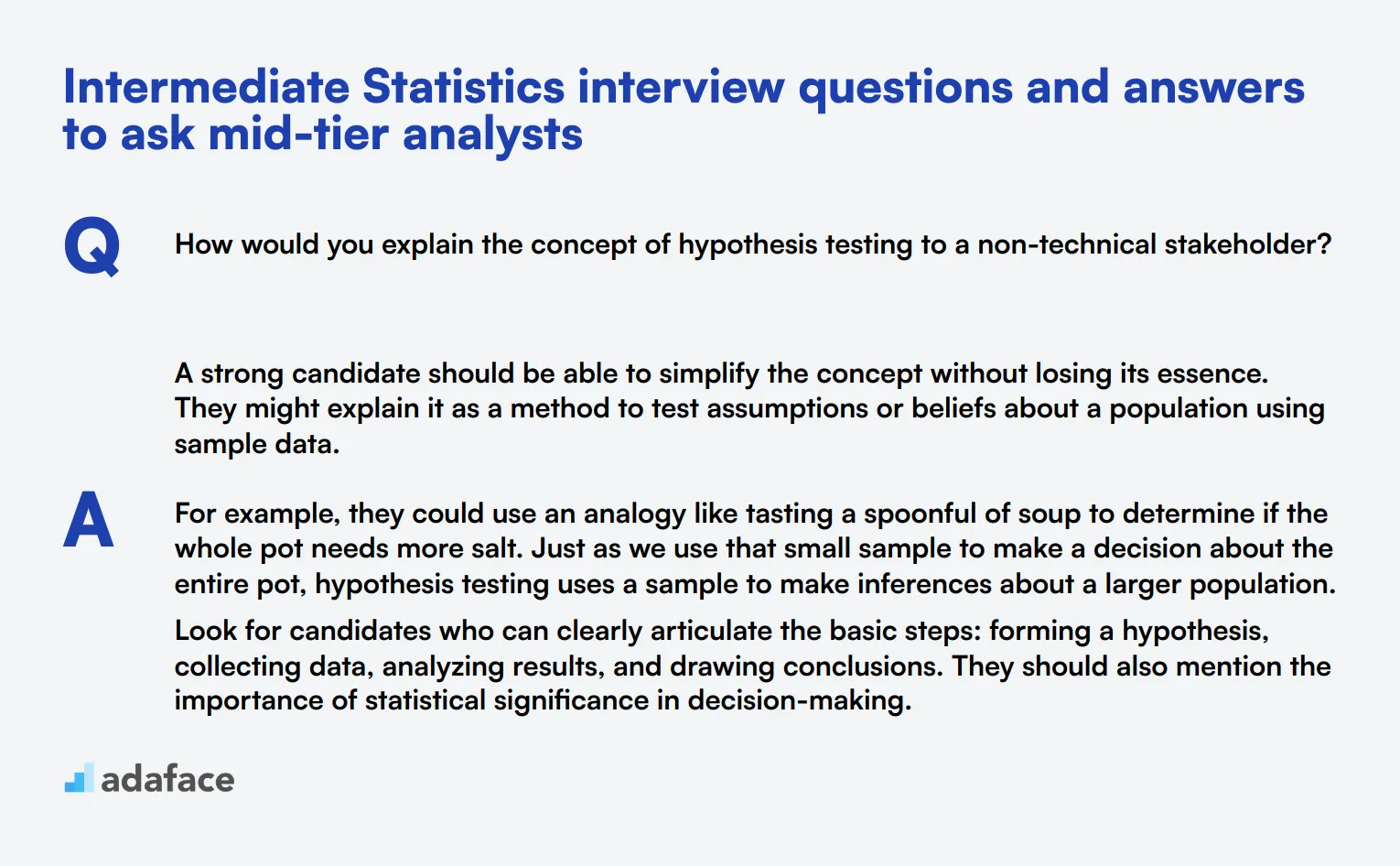
Ready to level up your statistics interviews? These 10 intermediate questions are perfect for assessing mid-tier analysts. They'll help you gauge a candidate's statistical know-how without getting too technical. Use them to spark insightful discussions and uncover how well applicants can apply statistical concepts to real-world scenarios.
1. How would you explain the concept of hypothesis testing to a non-technical stakeholder?
A strong candidate should be able to simplify the concept without losing its essence. They might explain it as a method to test assumptions or beliefs about a population using sample data.
For example, they could use an analogy like tasting a spoonful of soup to determine if the whole pot needs more salt. Just as we use that small sample to make a decision about the entire pot, hypothesis testing uses a sample to make inferences about a larger population.
Look for candidates who can clearly articulate the basic steps: forming a hypothesis, collecting data, analyzing results, and drawing conclusions. They should also mention the importance of statistical significance in decision-making.
2. Can you describe a situation where you had to use probability in a real-world analysis?
An ideal response should demonstrate the candidate's ability to apply probability concepts to practical scenarios. They might describe using probability in risk assessment, predicting customer behavior, or forecasting project outcomes.
For instance, they could explain how they used probability distributions to estimate the likelihood of different sales scenarios for a new product launch. This might involve analyzing historical data, identifying relevant factors, and creating a model to predict various outcomes.
Pay attention to how well the candidate explains their thought process and methodology. Look for their ability to translate complex probability concepts into actionable insights for decision-makers.
3. How would you approach analyzing a dataset with significant outliers?
A strong answer should outline a systematic approach to handling outliers. The candidate might suggest the following steps:
- Identify the outliers using statistical methods or visualizations
- Investigate the cause of the outliers (data entry errors, genuine anomalies, etc.)
- Decide on an appropriate treatment method based on the nature of the outliers and the analysis goals
- Consider multiple analysis approaches with and without outliers to understand their impact
- Clearly communicate the presence of outliers and their treatment in the final analysis
Look for candidates who emphasize the importance of understanding the context of the data and the potential impact of outliers on the analysis. They should also mention the need to document and justify their decisions regarding outlier treatment.
4. Explain the difference between a t-test and an ANOVA. When would you use each?
A knowledgeable candidate should be able to distinguish between these two common statistical tests and explain their appropriate use cases.
They might explain that a t-test is used to compare the means of two groups, while ANOVA (Analysis of Variance) is used to compare means across three or more groups. For example, a t-test could be used to compare the average height of men vs. women, while ANOVA could be used to compare the average heights of people from different countries.
Look for candidates who can provide examples of when each test would be appropriate and discuss assumptions like normality and equal variances. They should also mention that ANOVA can be seen as an extension of the t-test for multiple groups.
5. How would you explain the concept of statistical power to a project manager?
A strong response should demonstrate the ability to communicate complex statistical concepts in simple terms. The candidate might explain statistical power as the likelihood of detecting a real effect or difference when it exists.
They could use an analogy, such as comparing it to the sensitivity of a metal detector. Just as a more powerful metal detector can find smaller pieces of metal, a study with higher statistical power is more likely to detect smaller but meaningful effects in the data.
Look for candidates who emphasize the practical implications of statistical power, such as its impact on sample size determination and the interpretation of study results. They should also mention factors that influence power, like effect size and significance level.
6. Can you explain the concept of multicollinearity and its implications in regression analysis?
A competent candidate should be able to define multicollinearity as the high correlation between independent variables in a regression model. They should explain that while some correlation is expected, excessive multicollinearity can lead to unstable and unreliable coefficient estimates.
They might provide examples of how multicollinearity can occur, such as including both age and years of work experience in a model predicting salary. The candidate should discuss methods to detect multicollinearity, like variance inflation factor (VIF) or correlation matrices.
Look for responses that address the implications of multicollinearity, such as difficulty in determining the individual impact of variables and potential overfitting. Candidates should also mention strategies for dealing with multicollinearity, like removing redundant variables or using regularization techniques.
7. How would you go about choosing an appropriate sample size for a study?
A strong answer should outline a systematic approach to sample size determination. The candidate might mention the following factors to consider:
- Desired confidence level
- Margin of error
- Expected variability in the population
- Available resources (time, budget)
- Type of study (e.g., survey, clinical trial)
- Expected effect size
- Power analysis for hypothesis tests
Look for candidates who emphasize the balance between statistical rigor and practical constraints. They should mention tools or methods for calculating sample size, such as power analysis software or statistical formulas. A good response might also include a discussion of the trade-offs between larger and smaller sample sizes.
8. Can you explain the difference between precision and accuracy in the context of measurement and statistics?
A knowledgeable candidate should be able to clearly differentiate between precision and accuracy. They might explain that accuracy refers to how close a measurement is to the true value, while precision refers to the consistency or reproducibility of measurements.
They could use an analogy, such as archery. An accurate archer hits close to the bullseye on average, while a precise archer has tightly grouped shots (even if they're not necessarily close to the bullseye). An archer who is both accurate and precise would have tightly grouped shots near the bullseye.
Look for candidates who can discuss the implications of precision and accuracy in statistical analysis, such as their impact on measurement error and the reliability of results. They should also mention that high precision doesn't necessarily imply high accuracy, and vice versa.
9. How would you explain the concept of regression to the mean to a non-technical audience?
A strong response should demonstrate the ability to simplify complex statistical concepts. The candidate might explain regression to the mean as the tendency for extreme observations to move closer to the average in subsequent measurements.
They could use relatable examples, such as an athlete's performance over a season. If a player has an exceptionally good game, it's more likely their next game will be closer to their average performance. Similarly, after a particularly poor game, their next performance is likely to improve towards their usual level.
Look for candidates who can explain why this phenomenon occurs (random variation) and its implications in various fields, such as healthcare, education, or business. They should also mention how regression to the mean can lead to misinterpretation of data if not properly understood.
10. Can you describe a situation where you had to use bootstrapping in your analysis? What were the advantages and limitations?
An ideal response should demonstrate practical experience with bootstrapping techniques. The candidate might describe using bootstrapping to estimate confidence intervals for a statistic when the underlying distribution was unknown or when sample sizes were small.
They should explain that bootstrapping involves repeatedly resampling the original data with replacement to create multiple datasets, calculating the statistic of interest for each, and then using the distribution of these statistics to make inferences.
Look for candidates who can discuss both the advantages (e.g., no assumptions about underlying distributions, works well with small samples) and limitations (e.g., computationally intensive, sensitive to outliers) of bootstrapping. They should also be able to compare bootstrapping to other methods and explain when it's most appropriate to use.
15 advanced Statistics interview questions to ask senior analysts
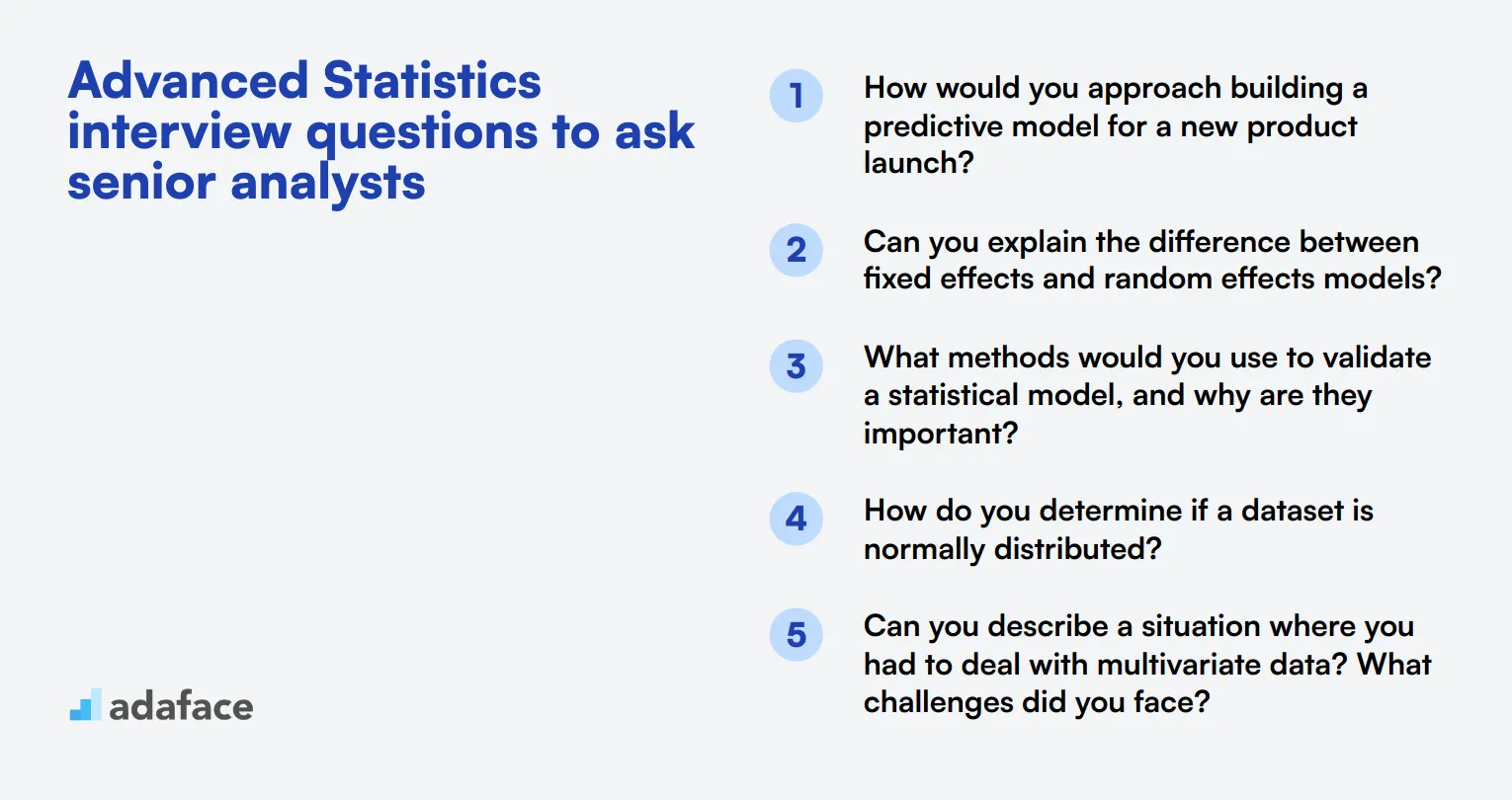
To ensure your candidates possess the advanced skills necessary for a senior statistics role, utilize this list of targeted interview questions. These inquiries can help you gauge their analytical abilities and practical experience, ultimately guiding you in making informed hiring decisions. For a more comprehensive understanding of potential roles, check out our Data Scientist job description.
- How would you approach building a predictive model for a new product launch?
- Can you explain the difference between fixed effects and random effects models?
- What methods would you use to validate a statistical model, and why are they important?
- How do you determine if a dataset is normally distributed?
- Can you describe a situation where you had to deal with multivariate data? What challenges did you face?
- What techniques would you use to assess the goodness of fit for a model?
- How do you decide on the appropriate statistical test when analyzing data?
- Can you explain how to interpret interaction effects in a regression model?
- What steps would you take to perform time series analysis, and what are key considerations?
- How do you ensure your statistical findings are reproducible?
- Can you describe a time when you had to communicate complex statistical results to a non-technical audience?
- What role does Bayesian statistics play in your analysis approach?
- How would you approach feature selection for a machine learning model?
- What are the practical implications of overfitting a model, and how can it be avoided?
- Can you explain how you would use AIC or BIC to compare models?
8 Statistics interview questions and answers related to probability theory
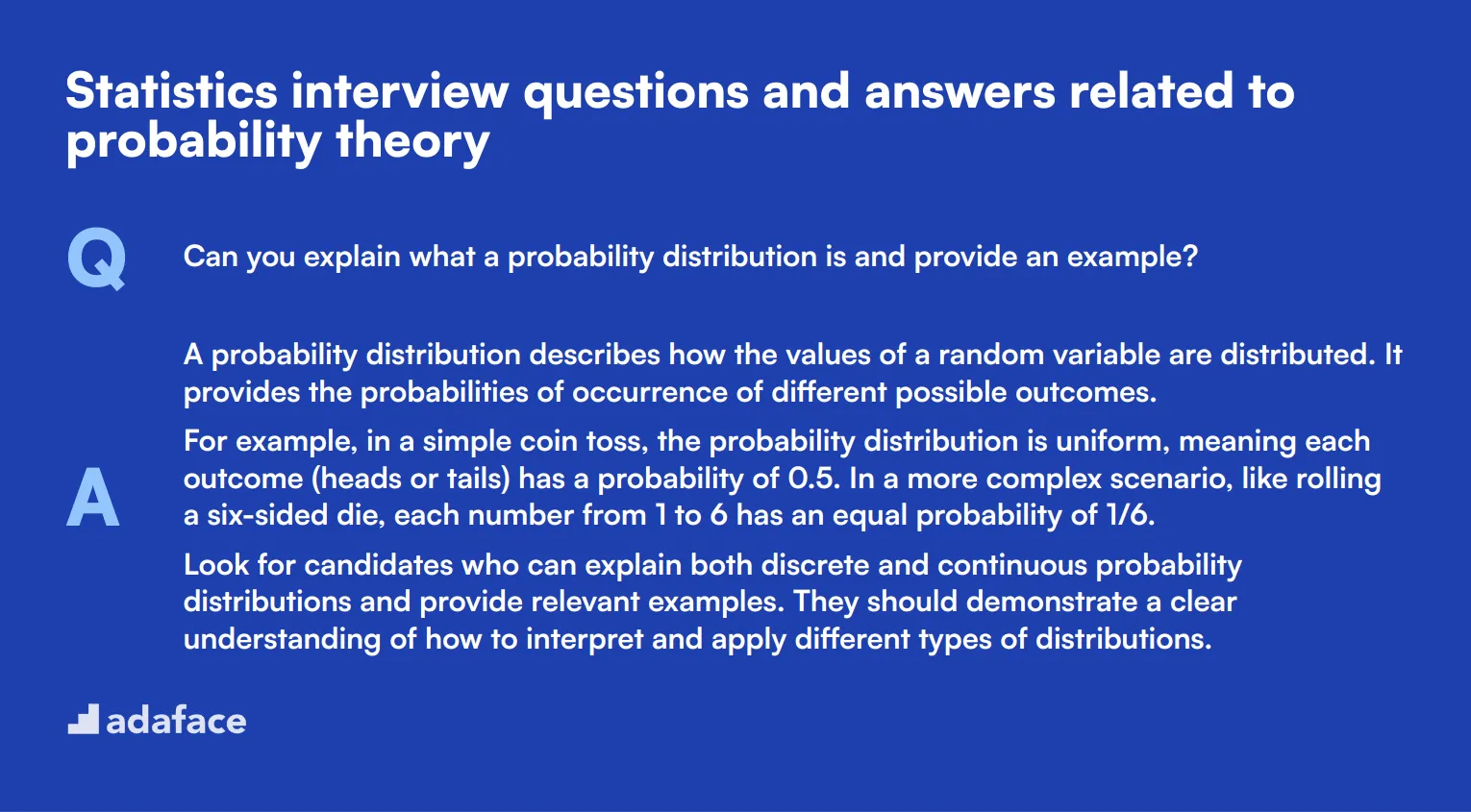
To determine whether your candidates have a solid grasp of probability theory, use these interview questions. These questions are designed to address key concepts and ensure your applicants can apply probability theory effectively in real-world scenarios.
1. Can you explain what a probability distribution is and provide an example?
A probability distribution describes how the values of a random variable are distributed. It provides the probabilities of occurrence of different possible outcomes.
For example, in a simple coin toss, the probability distribution is uniform, meaning each outcome (heads or tails) has a probability of 0.5. In a more complex scenario, like rolling a six-sided die, each number from 1 to 6 has an equal probability of 1/6.
Look for candidates who can explain both discrete and continuous probability distributions and provide relevant examples. They should demonstrate a clear understanding of how to interpret and apply different types of distributions.
2. What is the law of large numbers and why is it important?
The law of large numbers states that as the number of trials in a probability experiment increases, the average of the results will get closer to the expected value. Essentially, the more you repeat an experiment, the more the average outcome will resemble the theoretical probability.
This principle is crucial in fields like statistics and gambling, where it ensures that the results of many trials will stabilize around the expected value, giving a more accurate picture of the underlying probabilities.
Candidates should be able to illustrate the concept with examples and explain its significance in ensuring reliable outcomes in probability experiments. They should also mention its relevance in real-world applications such as insurance and finance.
3. How would you explain Bayes' Theorem to someone with no background in statistics?
Bayes' Theorem is a way of finding a probability when you know certain other probabilities. It describes the likelihood of an event, based on prior knowledge of conditions that might be related to the event.
For example, let's say you want to determine the probability of having a disease given a positive test result. Bayes' Theorem helps you calculate this by considering the overall probability of having the disease and the probability of testing positive if you have the disease.
An ideal candidate will break down Bayes' Theorem into simple, understandable parts and use relatable examples to demonstrate its application. They should emphasize its importance in fields like medicine, where it helps in diagnostic testing.
4. What is conditional probability and how is it different from regular probability?
Conditional probability is the probability of an event occurring given that another event has already occurred. It is denoted as P(A|B), meaning the probability of event A occurring given that event B has occurred.
For example, if you want to find out the probability of drawing an ace from a deck of cards, given that you've already drawn a king, you would use conditional probability. The regular probability of drawing an ace is 4/52, but the conditional probability changes to 4/51 because one card has already been drawn.
Candidates should clearly articulate the difference between conditional and regular probability and provide practical examples. They should demonstrate an understanding of how conditional probability is used in various scenarios, such as risk assessment and decision-making processes.
5. Can you explain what a random variable is?
A random variable is a variable whose possible values are numerical outcomes of a random phenomenon. There are two types of random variables: discrete and continuous.
For example, in a dice roll, the outcome (1 through 6) is a discrete random variable, whereas the time it takes for a radioactive particle to decay can be considered a continuous random variable.
Look for candidates who can clearly define both types of random variables and provide relevant examples. They should also explain how random variables are used in statistical analysis to model and understand random processes.
6. How would you apply the concept of expected value in decision-making?
Expected value is a measure of the center of a probability distribution and represents the average outcome if an experiment is repeated many times. It is calculated by multiplying each possible outcome by its probability and summing the results.
In decision-making, expected value helps to identify the most beneficial option. For instance, in a business scenario, if you have to choose between two investment options, calculating the expected value of the returns can guide you to the better choice.
Candidates should explain the calculation of expected value and provide real-world examples of its application, such as in financial investments or risk management. They should demonstrate an ability to use expected value for making informed decisions.
7. What is the difference between independent and mutually exclusive events?
Independent events are those whose occurrence does not affect the probability of the other event occurring. For example, flipping a coin and rolling a die are independent events because the outcome of one does not influence the outcome of the other.
Mutually exclusive events, on the other hand, cannot happen at the same time. For instance, in a single dice roll, getting a 2 and getting a 3 are mutually exclusive events. If one occurs, the other cannot.
An ideal candidate will clearly distinguish between these two types of events and provide relevant examples. They should also explain how understanding these concepts is crucial in probability calculations and real-world scenarios.
8. How do you interpret the probability of an event that is close to 0 or 1?
The probability of an event ranges from 0 to 1, where 0 means the event will not happen and 1 means the event will certainly happen. If the probability of an event is close to 0, it is very unlikely to occur. Conversely, if it is close to 1, it is very likely to occur.
For example, the probability of flipping a coin and it landing on heads is 0.5, but if you say the probability of it landing on heads 100 times in a row is close to 0, it means it's almost impossible.
Candidates should demonstrate an understanding of how to interpret these probabilities and provide practical examples. They should also discuss how probabilities are used to make predictions and assess risks in various fields.
8 Statistics interview questions and answers related to regression analysis
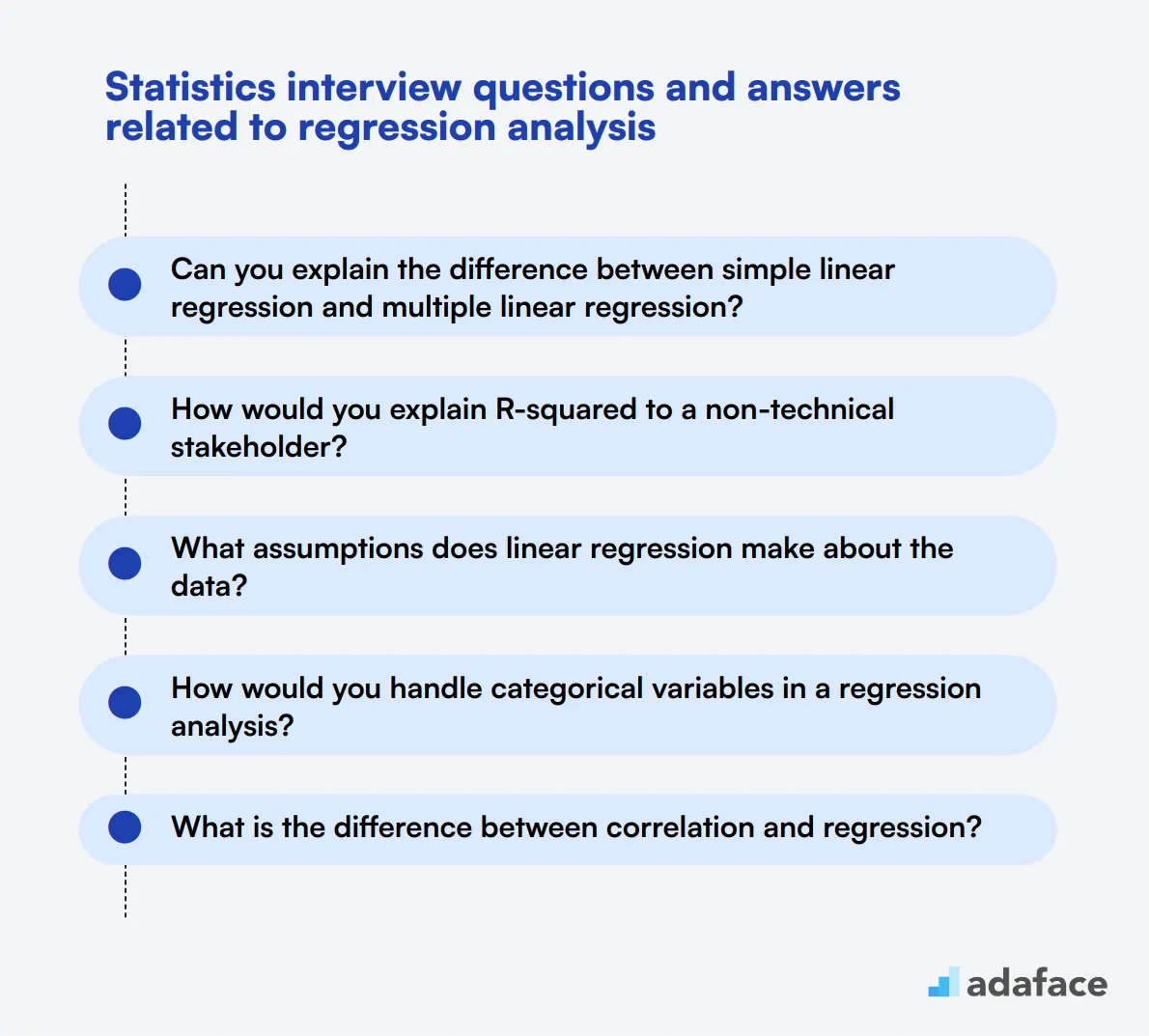
Ready to dive into the world of regression analysis? These 8 interview questions will help you assess candidates' understanding of this crucial statistical technique. Whether you're hiring for a data scientist or a business analyst role, these questions will give you insight into how well applicants can apply regression concepts in real-world scenarios.
1. Can you explain the difference between simple linear regression and multiple linear regression?
Simple linear regression involves one independent variable and one dependent variable, while multiple linear regression involves two or more independent variables and one dependent variable.
In simple linear regression, we're trying to fit a straight line to the data points, whereas in multiple linear regression, we're fitting a hyperplane in a multidimensional space.
Look for candidates who can clearly articulate the difference and provide examples of when each type might be used. Strong candidates might also mention the increased complexity of interpreting results in multiple regression due to potential interactions between variables.
2. How would you explain R-squared to a non-technical stakeholder?
R-squared is a statistical measure that represents the proportion of the variance in the dependent variable that is predictable from the independent variable(s). It's often described as the 'goodness of fit' measure for linear regression models.
To explain it to a non-technical stakeholder, you might say: "R-squared tells us how well our model explains the data. It ranges from 0 to 1, where 0 means our model doesn't explain any of the variability in the data, and 1 means it explains all of the variability. For example, an R-squared of 0.7 means our model explains 70% of the variability in the outcome we're trying to predict."
Look for candidates who can simplify complex concepts without losing accuracy. They should be able to use analogies or real-world examples to make the explanation more relatable.
3. What assumptions does linear regression make about the data?
Linear regression makes several key assumptions about the data:
- Linearity: The relationship between the independent and dependent variables is linear.
- Independence: The observations are independent of each other.
- Homoscedasticity: The variance of residual is the same for any value of X.
- Normality: The residuals are normally distributed.
- No or little multicollinearity: The independent variables are not highly correlated with each other.
Strong candidates should be able to not only list these assumptions but also explain why they're important and how to check for violations. They might also discuss the consequences of violating these assumptions and potential remedies.
4. How would you handle categorical variables in a regression analysis?
Handling categorical variables in regression analysis typically involves converting them into numerical form through a process called encoding. The two main methods are:
- Dummy Encoding (One-Hot Encoding): Creating binary columns for each category, where 1 indicates presence and 0 indicates absence.
- Effect Coding: Similar to dummy coding, but uses -1, 0, and 1 to represent categories.
Look for candidates who understand the pros and cons of each method. They should mention that dummy encoding can lead to the 'dummy variable trap' if not handled properly, and discuss when to use each method. Advanced candidates might also mention other techniques like feature hashing or embedding for high-cardinality categorical variables.
5. What is the difference between correlation and regression?
Correlation measures the strength and direction of a linear relationship between two variables. It ranges from -1 to 1, where -1 is a perfect negative correlation, 0 is no correlation, and 1 is a perfect positive correlation. Regression, on the other hand, is used to predict the value of a dependent variable based on the value of one or more independent variables.
While correlation tells us if there's a relationship between variables, regression allows us to quantify that relationship and make predictions. For example, correlation might tell us that height and weight are related, while regression would allow us to predict weight given a person's height.
Strong candidates should be able to explain that correlation doesn't imply causation, while regression can be used to model causal relationships under certain conditions. They might also discuss how regression can be used with multiple variables, while correlation is typically used with just two.
6. How do you interpret the coefficients in a multiple regression model?
In a multiple regression model, each coefficient represents the change in the dependent variable for a one-unit change in the corresponding independent variable, holding all other variables constant. For example, if we have a model predicting salary based on years of experience and education level, a coefficient of 5000 for years of experience would mean that, on average, each additional year of experience is associated with a $5000 increase in salary, assuming education level remains constant.
It's important to note that the interpretation assumes the model is correctly specified and all other variables are held constant. The intercept represents the expected value of the dependent variable when all independent variables are zero.
Look for candidates who can explain this clearly and also discuss the importance of statistical significance when interpreting coefficients. Strong candidates might also mention standardized coefficients and how they can be used to compare the relative importance of different variables in the model.
7. What is multicollinearity and why is it a problem in regression analysis?
Multicollinearity occurs when two or more independent variables in a regression model are highly correlated with each other. This can lead to several problems:
- Unstable and unreliable coefficient estimates
- Inflated standard errors
- Difficulty in determining the individual importance of a predictor
- Overfitting of the model
Look for candidates who can explain why multicollinearity is problematic and how to detect it (e.g., using Variance Inflation Factor or correlation matrices). Strong candidates might also discuss methods to address multicollinearity, such as removing one of the correlated variables, combining variables, or using regularization techniques like ridge regression.
8. How would you validate the assumptions of a regression model?
Validating the assumptions of a regression model typically involves several steps:
- Linearity: Plot residuals vs. predicted values to check for any patterns.
- Independence: Use Durbin-Watson test or plot residuals against time/order.
- Homoscedasticity: Use Breusch-Pagan test or plot residuals vs. predicted values.
- Normality: Use Q-Q plots or statistical tests like Shapiro-Wilk.
- Multicollinearity: Calculate Variance Inflation Factors (VIF) or correlation matrix.
Strong candidates should be able to explain these methods and interpret their results. They might also discuss the importance of visual inspection alongside statistical tests. Look for candidates who understand that minor violations of assumptions might be acceptable, but severe violations require addressing through data transformations or alternative modeling approaches.
9 situational Statistics interview questions with answers for hiring top analysts
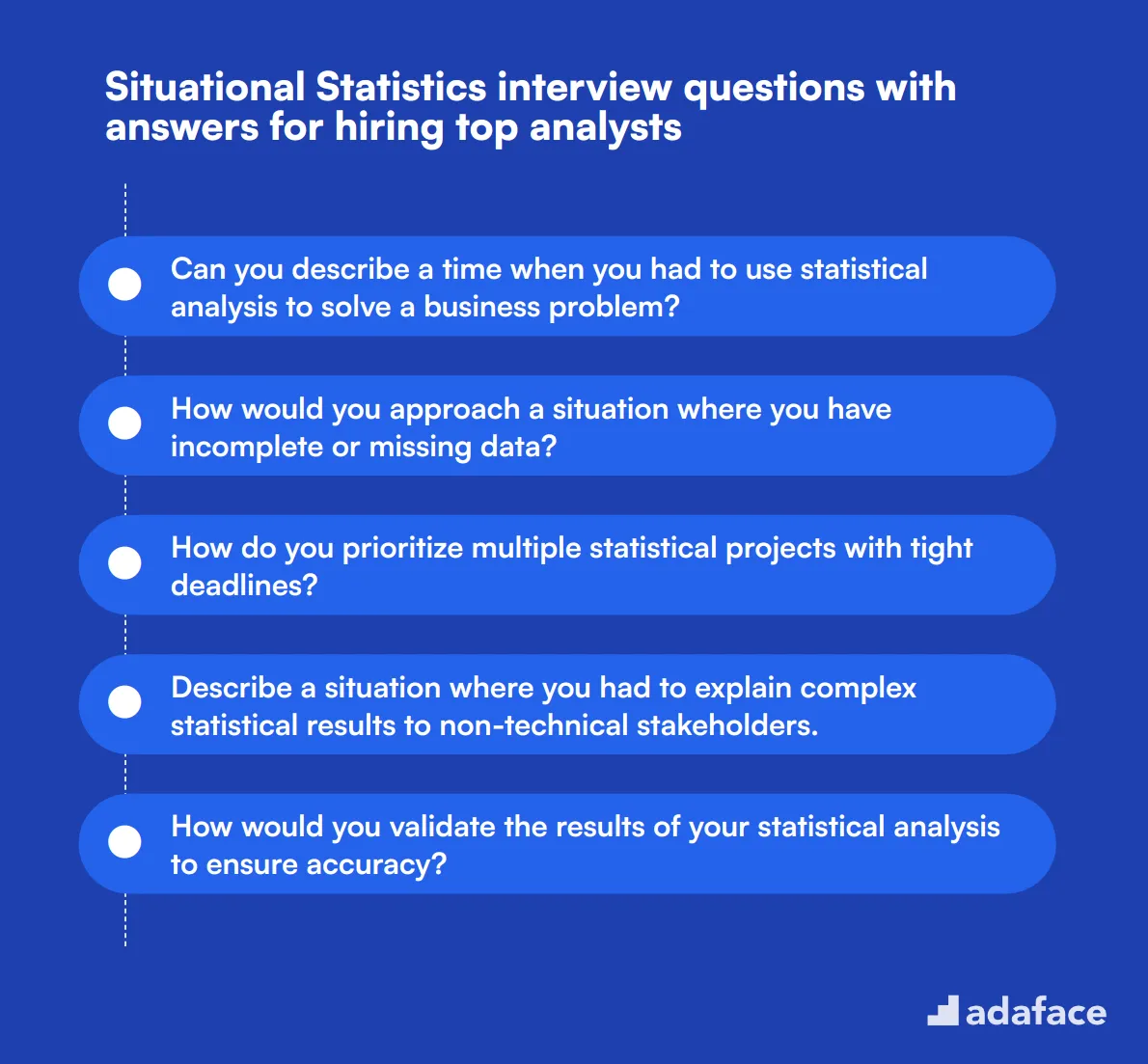
To find the top analysts for your team, it’s crucial to ask the right situational questions during interviews. This list of situational statistics interview questions will help you evaluate candidates’ problem-solving abilities and how they apply their statistical knowledge in real-world scenarios.
1. Can you describe a time when you had to use statistical analysis to solve a business problem?
A strong candidate will provide a specific example of a business problem they faced and how they used statistical analysis to solve it. They should outline the problem, the data they analyzed, the statistical methods they employed, and the outcomes of their analysis.
Look for candidates who can clearly explain their thought process and demonstrate how their statistical expertise directly contributed to solving the business problem. It's a bonus if they can provide metrics or quantifiable results from their analysis.
2. How would you approach a situation where you have incomplete or missing data?
Handling incomplete or missing data is a common challenge in statistics. An ideal candidate would first assess the extent and pattern of the missing data. They might mention techniques such as imputation, where missing values are replaced based on other available data, or using algorithms that can handle missing data directly.
The candidate might also discuss the importance of understanding why the data is missing and considering the potential impact on the analysis. They should highlight the importance of maintaining data integrity and how to minimize bias in their results.
Look for candidates who demonstrate a systematic approach to addressing missing data and can articulate the pros and cons of different methods.
3. How do you prioritize multiple statistical projects with tight deadlines?
Candidates should mention strategies for prioritization such as assessing the impact and urgency of each project, and breaking down projects into smaller, manageable tasks. They might also discuss using project management tools and techniques to keep track of deadlines and progress.
Strong answers will also include examples of how they have effectively managed their time and resources in past projects, and how they communicated with stakeholders to set realistic expectations.
Look for candidates who can demonstrate effective time management and the ability to balance multiple priorities without sacrificing quality.
4. Describe a situation where you had to explain complex statistical results to non-technical stakeholders.
An effective candidate should be able to provide a specific example where they successfully conveyed complex statistical results in a clear and understandable manner. They might mention using data visualization tools, simplifying jargon, or creating analogies to make the concepts more relatable.
The candidate should also emphasize the importance of understanding the audience's level of knowledge and tailoring their communication style accordingly. They should highlight any positive feedback or outcomes resulting from their explanation.
Look for candidates who can balance technical accuracy with simplicity and show a strong ability to communicate effectively with non-technical stakeholders.
5. How would you validate the results of your statistical analysis to ensure accuracy?
A thorough candidate would discuss multiple validation techniques such as cross-validation, using different datasets, or conducting sensitivity analyses. They might also mention peer reviews or seeking feedback from other experts to ensure robustness and accuracy.
The candidate should talk about the importance of checking assumptions and ensuring that the data meets the criteria required for the chosen statistical methods. They might also mention using software tools to automate and double-check calculations.
Look for candidates who emphasize a meticulous approach to validation and the importance of accuracy in their analyses. They should demonstrate a clear understanding of different validation methods and their applications.
6. Can you give an example of a time when you identified a flaw in a statistical model or analysis? How did you handle it?
A strong candidate will provide a specific example of identifying a flaw in a statistical model or analysis. They should describe the flaw, how they discovered it, and the steps they took to address and correct it.
The candidate might mention re-evaluating assumptions, conducting additional analyses, or consulting with colleagues to ensure the flaw was thoroughly understood and resolved. They should also discuss the importance of transparency and how they communicated the issue and resolution to stakeholders.
Look for candidates who demonstrate critical thinking, attention to detail, and a proactive approach to identifying and resolving issues in their analyses.
7. How do you stay updated with the latest trends and advancements in statistics?
Candidates should mention various ways they keep up-to-date, such as reading academic journals, attending conferences and webinars, participating in online forums and communities, or enrolling in continuing education courses.
They might also discuss following influential figures in the field on social media, subscribing to newsletters, or being part of professional organizations. The candidate should emphasize the importance of continuous learning and staying current with new methodologies and technologies.
Look for candidates who show a genuine passion for the field and a proactive approach to professional development and staying informed about the latest trends.
8. What methods do you use to ensure the data you collect is reliable and valid?
A solid candidate will discuss multiple methods to ensure data reliability and validity, such as using standardized data collection procedures, conducting pilot tests, and employing rigorous data cleaning techniques.
They might also mention cross-referencing data from multiple sources, ensuring consistency, and applying statistical tests to check for reliability and validity. The candidate should highlight the importance of accurate data collection in producing trustworthy and meaningful results.
Look for candidates who demonstrate a comprehensive understanding of data quality assurance and can articulate specific methods they use to ensure data reliability and validity.
9. How would you approach conducting a statistical analysis for a new, unfamiliar dataset?
Candidates should outline a clear and systematic approach to tackling a new dataset. They might start by understanding the context and objectives of the analysis, followed by exploring and cleaning the data to identify any issues or patterns.
The candidate should discuss selecting appropriate statistical methods and tools based on the dataset's characteristics and the analysis objectives. They might also mention conducting preliminary analyses and iteratively refining their approach as they gain more insights.
Look for candidates who demonstrate a methodical and thoughtful approach to new datasets, with an emphasis on understanding the data context and objectives before diving into the analysis.
Which Statistics skills should you evaluate during the interview phase?
While it's challenging to assess every aspect of a candidate's statistical prowess in a single interview, focusing on core skills is essential. Here are key statistical abilities to evaluate during the interview process to identify top-tier candidates.
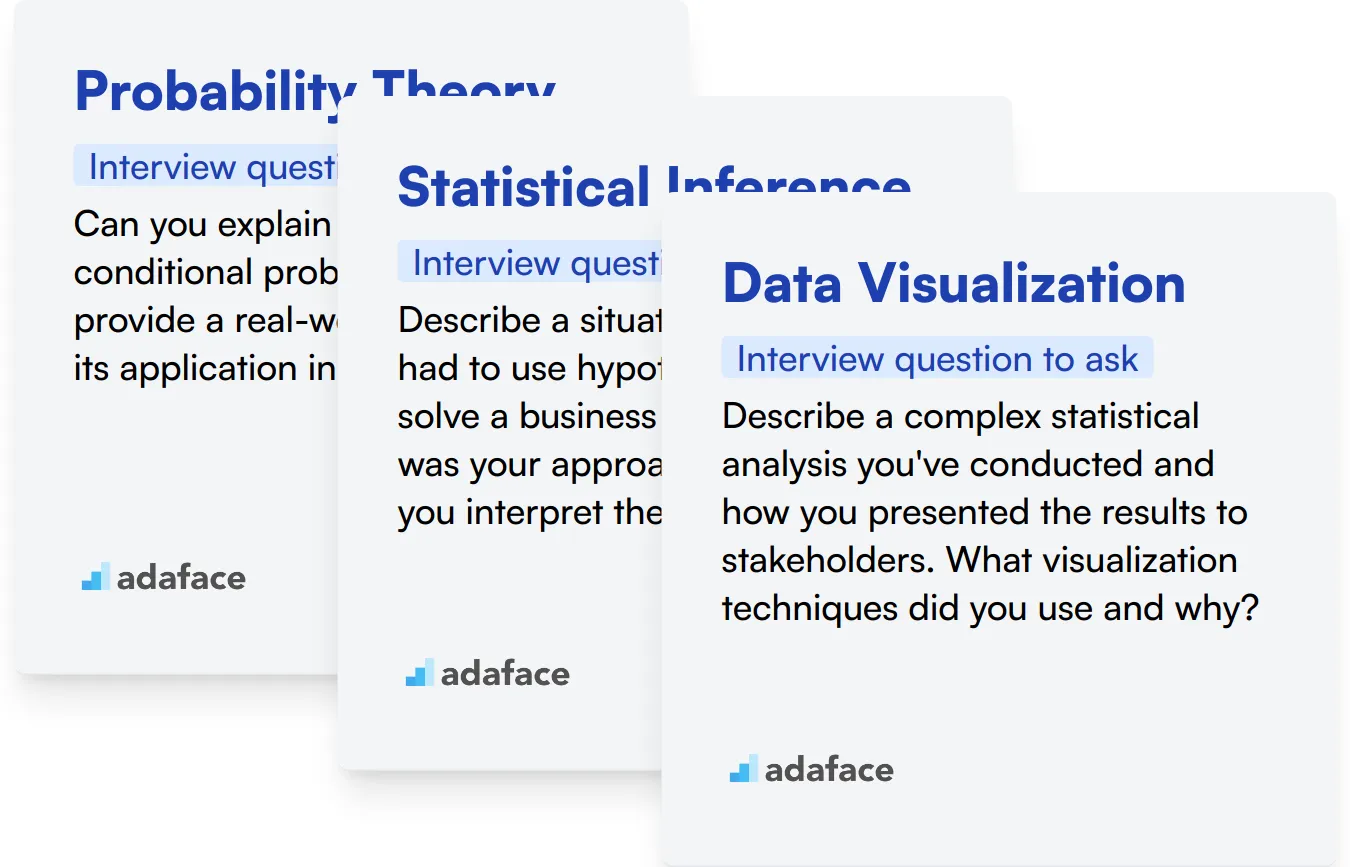
Probability Theory
Probability theory forms the backbone of statistical analysis. It enables analysts to quantify uncertainty and make informed predictions based on available data.
To assess this skill, consider using an assessment test with relevant multiple-choice questions on probability concepts. This can help filter candidates with strong foundational knowledge.
You can also ask targeted interview questions to gauge the candidate's understanding of probability theory. Here's an example question:
Can you explain the concept of conditional probability and provide a real-world example of its application in data analysis?
Look for answers that demonstrate a clear understanding of conditional probability. The candidate should be able to explain how it's calculated and provide a relevant example, such as its use in Bayesian inference or risk assessment.
Statistical Inference
Statistical inference is key to drawing meaningful conclusions from data. It involves making estimates about populations based on sample data and testing hypotheses.
To evaluate this skill, you might use an assessment test that includes questions on hypothesis testing, confidence intervals, and p-values. This can help identify candidates with strong inferential skills.
During the interview, you can ask questions that test the candidate's ability to apply statistical inference concepts. Here's an example:
Describe a situation where you had to use hypothesis testing to solve a business problem. What was your approach, and how did you interpret the results?
Look for responses that show the candidate can apply hypothesis testing in practical scenarios. They should be able to explain their choice of test, the null and alternative hypotheses, and how they interpreted the results in the context of the business problem.
Data Visualization
Data visualization is crucial for presenting statistical findings effectively. It helps in communicating complex data insights to both technical and non-technical audiences.
Consider using an assessment that includes questions on choosing appropriate charts and graphs for different types of data. This can help identify candidates who can effectively present statistical results.
To further assess this skill during the interview, you might ask:
Describe a complex statistical analysis you've conducted and how you presented the results to stakeholders. What visualization techniques did you use and why?
Look for answers that demonstrate the candidate's ability to choose appropriate visualization methods for different types of data and audiences. They should be able to explain how their visualizations enhanced understanding of the statistical findings.
Enhance Your Team with the Right Statistics Skills Tests and Interview Questions
If you're aiming to recruit individuals with proficient Statistics skills, it's imperative to confirm these abilities accurately. Ensuring candidates possess the necessary skill set grounds the basis for hiring.
The most direct method to gauge these skills is through targeted skills tests. Consider utilizing Adaface's Statistics Online Test or the Data Analysis Test to evaluate candidate capabilities effectively.
After deploying these tests, you can efficiently sift through the applicant pool to identify the top contenders. This streamlines the initial phase of the hiring process, allowing you to focus on interviewing the most promising candidates.
Ready to start the hiring process? Sign up on the Adaface Dashboard to access all the tools necessary for recruiting top-tier talent, or explore the test library to find the perfect assessment for your needs.
Statistics Test
Download Statistics interview questions template in multiple formats
Statistics Interview Questions FAQs
Focus on basic concepts and fundamental statistical techniques to gauge their foundational knowledge.
Ask questions related to probability distributions, theorems, and real-world applications of probability theory.
Present complex scenarios that require advanced problem-solving skills and strategic thinking in statistics.
Ask about different types of regression models, their applications, and how to interpret the results.
Yes, combining skills tests with interview questions can provide a comprehensive assessment of a candidate's abilities.
It ensures consistency, helps in comparing candidates objectively, and covers all necessary topics effectively.
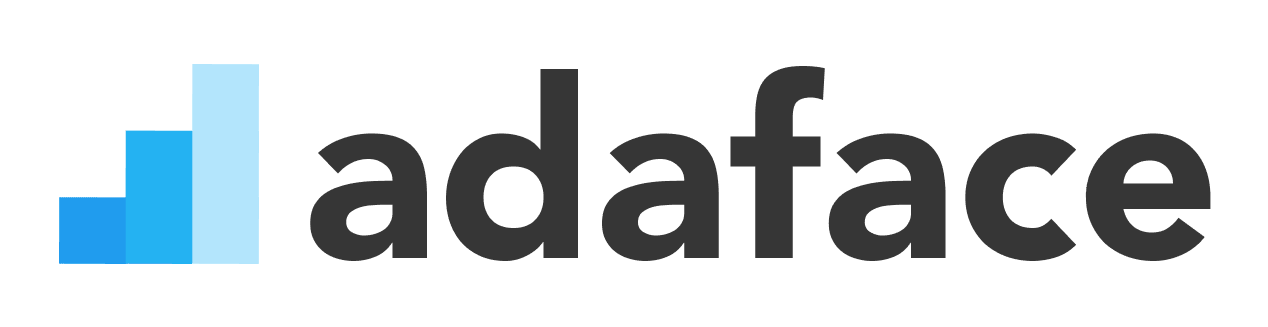
40 min skill tests.
No trick questions.
Accurate shortlisting.
We make it easy for you to find the best candidates in your pipeline with a 40 min skills test.
Try for freeRelated posts
Free resources
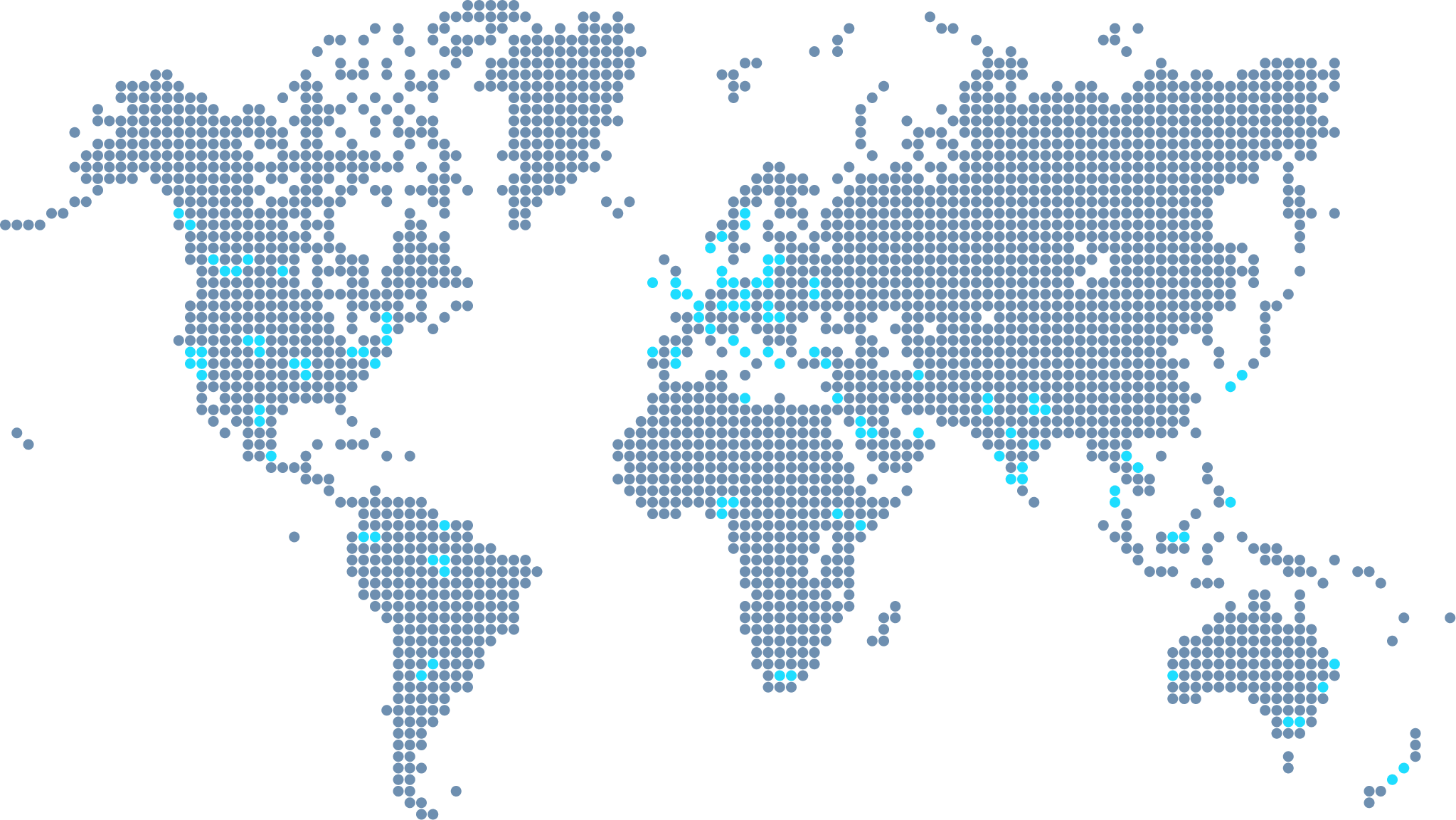
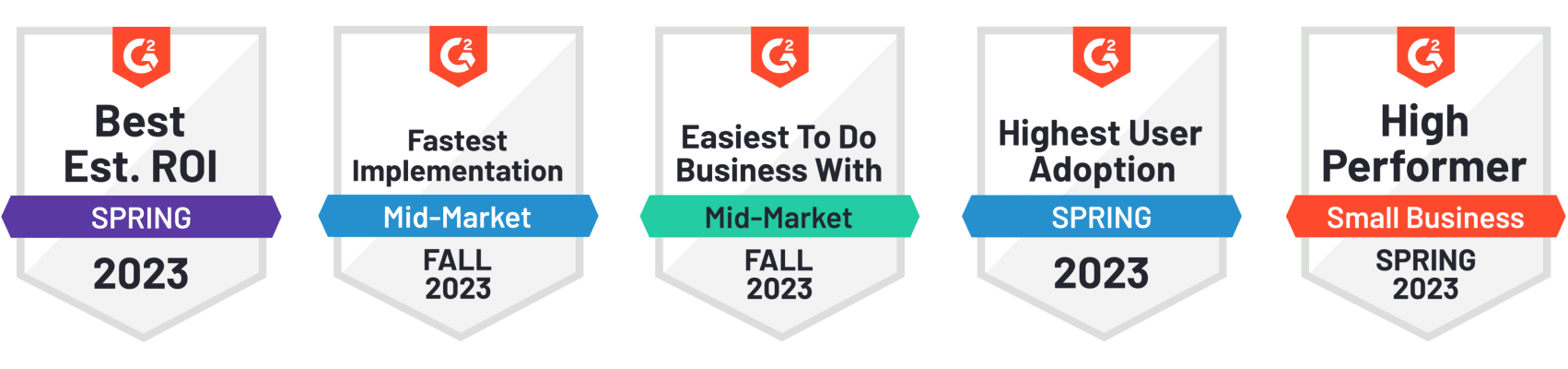