Interviewing candidates for roles requiring quantitative skills can be challenging without a targeted approach. Knowing what questions to ask is important to identify candidates with the right level of expertise for your team.
This blog post offers a comprehensive list of quantitative skills interview questions tailored for different experience levels. Whether you’re hiring junior analysts or senior professionals, we’ve got you covered.
Use this guide to fine-tune your interview process and ensure you hire the best candidates. For a more thorough evaluation, consider our quantitative aptitude test as a pre-interview screening tool.
Table of contents
Top 8 Quantitative Skills questions to ask in interviews
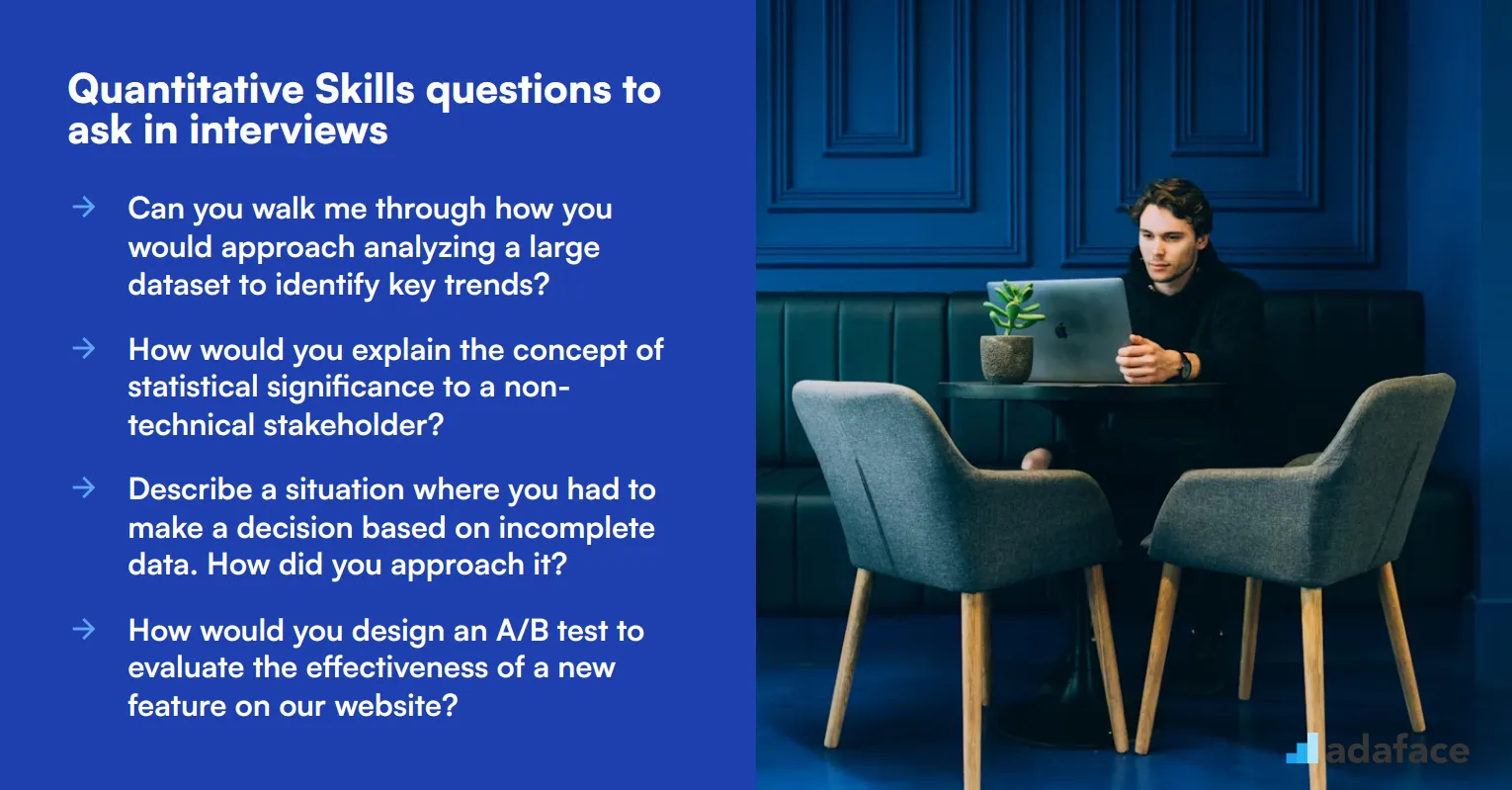
Ready to uncover the quantitative wizards in your candidate pool? These eight carefully crafted questions will help you assess quantitative skills effectively during interviews. Use them to gauge analytical thinking, problem-solving abilities, and numerical proficiency. Remember, the goal is to understand how candidates approach quantitative challenges, not just whether they can crunch numbers.
1. Can you walk me through how you would approach analyzing a large dataset to identify key trends?
A strong candidate should outline a structured approach to data analysis. They might mention steps such as:
- Understanding the context and objectives of the analysis
- Cleaning and preprocessing the data
- Exploring the data through descriptive statistics and visualizations
- Applying appropriate statistical techniques or machine learning algorithms
- Interpreting results and drawing actionable insights
- Communicating findings effectively to stakeholders
Look for candidates who emphasize the importance of understanding the business context, mention specific tools or techniques they've used, and discuss how they would validate their findings. A good follow-up question might be to ask for an example of a challenging dataset they've worked with in the past.
2. How would you explain the concept of statistical significance to a non-technical stakeholder?
An ideal response should demonstrate the candidate's ability to simplify complex concepts. They might use an analogy or real-world example, such as:
"Imagine we're testing a new medicine. Statistical significance is like having enough evidence to be confident that the medicine's effects aren't just due to chance. It's our way of saying, 'We're pretty sure this medicine is actually doing something, and it's not just random luck.' The more data we have, the more confident we can be."
Look for candidates who can break down the concept without using technical jargon. They should touch on the ideas of probability, sample size, and the balance between Type I and Type II errors, even if they don't use these exact terms. Consider asking how they would determine an appropriate significance level for different types of business decisions.
3. Describe a situation where you had to make a decision based on incomplete data. How did you approach it?
This question assesses a candidate's ability to handle uncertainty and make data-driven decisions in real-world scenarios. A strong answer might include:
- Identifying the key missing information and its potential impact
- Using available data to make reasonable assumptions or estimates
- Applying sensitivity analysis to understand how different scenarios might affect the outcome
- Clearly communicating the limitations and risks associated with the decision
- Proposing a plan to gather additional data or validate assumptions over time
Pay attention to how candidates balance analytical thinking with practical decision-making. Look for those who can articulate their thought process and demonstrate how they mitigate risks when working with imperfect information. You might follow up by asking how they would improve their approach if faced with a similar situation in the future.
4. How would you design an A/B test to evaluate the effectiveness of a new feature on our website?
A comprehensive answer should cover the key steps in designing and executing an A/B test:
- Defining clear objectives and success metrics
- Determining the appropriate sample size and test duration
- Randomly assigning users to control and treatment groups
- Implementing the test while minimizing external factors
- Collecting and analyzing data using appropriate statistical methods
- Drawing conclusions and recommending next steps based on results
Look for candidates who emphasize the importance of statistical power in test design and mention potential pitfalls like selection bias or the multiple comparison problem. A good follow-up question might be to ask how they would handle a situation where the test results are inconclusive.
5. If our company's revenue has increased by 5% year-over-year, but inflation is at 7%, how would you interpret this data?
This question tests the candidate's ability to contextualize financial data. A strong answer should include:
- Recognition that the 5% increase in revenue is in nominal terms
- Calculation of the real revenue growth: approximately -2% (5% - 7%)
- Interpretation that the company's purchasing power has actually decreased
- Consideration of other factors that might affect this interpretation, such as industry trends or company-specific circumstances
Look for candidates who can quickly perform mental math and provide insights beyond the surface-level numbers. They should be able to explain the concept of real vs. nominal growth and discuss potential implications for the business. You might follow up by asking how they would recommend addressing this situation in a business context.
6. Explain the difference between correlation and causation, and provide an example where mistaking one for the other could lead to poor business decisions.
A strong response should clearly differentiate between correlation (a statistical relationship between two variables) and causation (one variable directly influencing another). An example might be:
"Imagine an ice cream shop notices that their sales increase on days when more people wear sunglasses. While there's a correlation between sunglasses and ice cream sales, assuming causation might lead the shop to start giving out free sunglasses to boost sales. In reality, both factors are likely caused by sunny weather, and the sunglasses promotion wouldn't necessarily increase sales."
Look for candidates who can articulate the importance of considering confounding variables and conducting controlled experiments to establish causation. They should be able to discuss how misinterpreting correlation as causation can lead to misallocation of resources or ineffective strategies. Consider asking how they would approach determining causation in a business context.
7. How would you approach forecasting sales for a new product with no historical data?
This question assesses the candidate's ability to make predictions with limited information. A comprehensive answer might include:
- Analyzing sales data from similar products or product categories
- Researching market trends and competitor performance
- Conducting customer surveys or focus groups to gauge interest
- Using analogous estimating techniques from other industries or markets
- Developing multiple scenarios (best case, worst case, most likely)
- Applying statistical techniques like Monte Carlo simulation to account for uncertainty
Look for candidates who emphasize the importance of clearly stating assumptions and continuously refining forecasts as new data becomes available. They should be able to discuss the limitations of their approach and propose methods for validating their predictions over time. A good follow-up question might be to ask how they would adjust their forecast if early sales data differs significantly from predictions.
8. If you had to reduce our company's expenses by 10%, how would you approach this task from a data analysis perspective?
A strong answer should outline a systematic, data-driven approach to cost reduction:
- Gathering comprehensive data on all company expenses
- Categorizing and analyzing expenses by department, type, and necessity
- Identifying trends and anomalies in spending patterns
- Benchmarking against industry standards or competitors
- Performing sensitivity analysis to understand the impact of different cost-cutting scenarios
- Prioritizing cuts based on potential savings and impact on operations
- Proposing a phased implementation plan with clear metrics for success
Look for candidates who emphasize the importance of collaborating with different departments and considering both short-term savings and long-term implications. They should be able to discuss how they would use data visualization techniques to communicate findings effectively to stakeholders. Consider asking how they would handle potential resistance to their recommendations or how they would validate the effectiveness of implemented cost-cutting measures.
20 Quantitative Skills interview questions to ask junior analysts
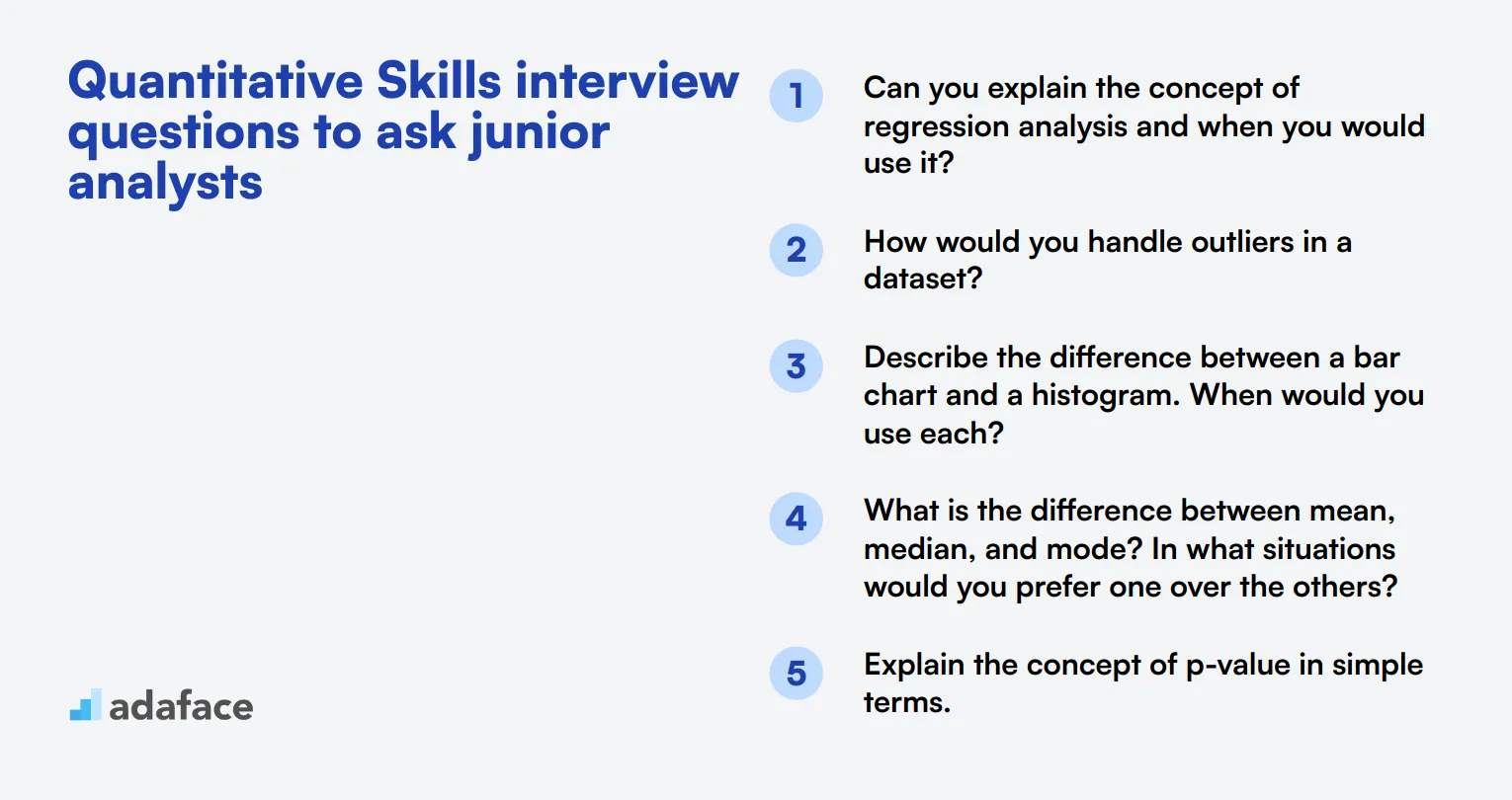
When interviewing junior analysts, it's crucial to assess their quantitative skills. These 20 questions will help you evaluate candidates' analytical abilities, problem-solving skills, and technical knowledge. Use them to gauge how well applicants can handle real-world data challenges in your organization.
- Can you explain the concept of regression analysis and when you would use it?
- How would you handle outliers in a dataset?
- Describe the difference between a bar chart and a histogram. When would you use each?
- What is the difference between mean, median, and mode? In what situations would you prefer one over the others?
- Explain the concept of p-value in simple terms.
- How would you approach cleaning a dataset with missing values?
- What is the difference between a population and a sample? Why is this distinction important?
- Can you explain what a confidence interval is and how you would interpret it?
- How would you detect and address multicollinearity in a regression model?
- Explain the concept of Type I and Type II errors in hypothesis testing.
- What is the purpose of data normalization, and when would you use it?
- How would you approach analyzing time series data?
- Explain the difference between supervised and unsupervised learning in machine learning.
- What is the purpose of cross-validation in model evaluation?
- How would you handle imbalanced data in a classification problem?
- Explain the concept of dimensionality reduction and why it's important in data analysis.
- What is the difference between parametric and non-parametric statistical tests?
- How would you approach feature selection for a predictive model?
- Explain the concept of overfitting and how you would prevent it.
- What is the difference between correlation and covariance? When would you use each?
10 intermediate Quantitative Skills interview questions and answers to ask mid-tier analysts
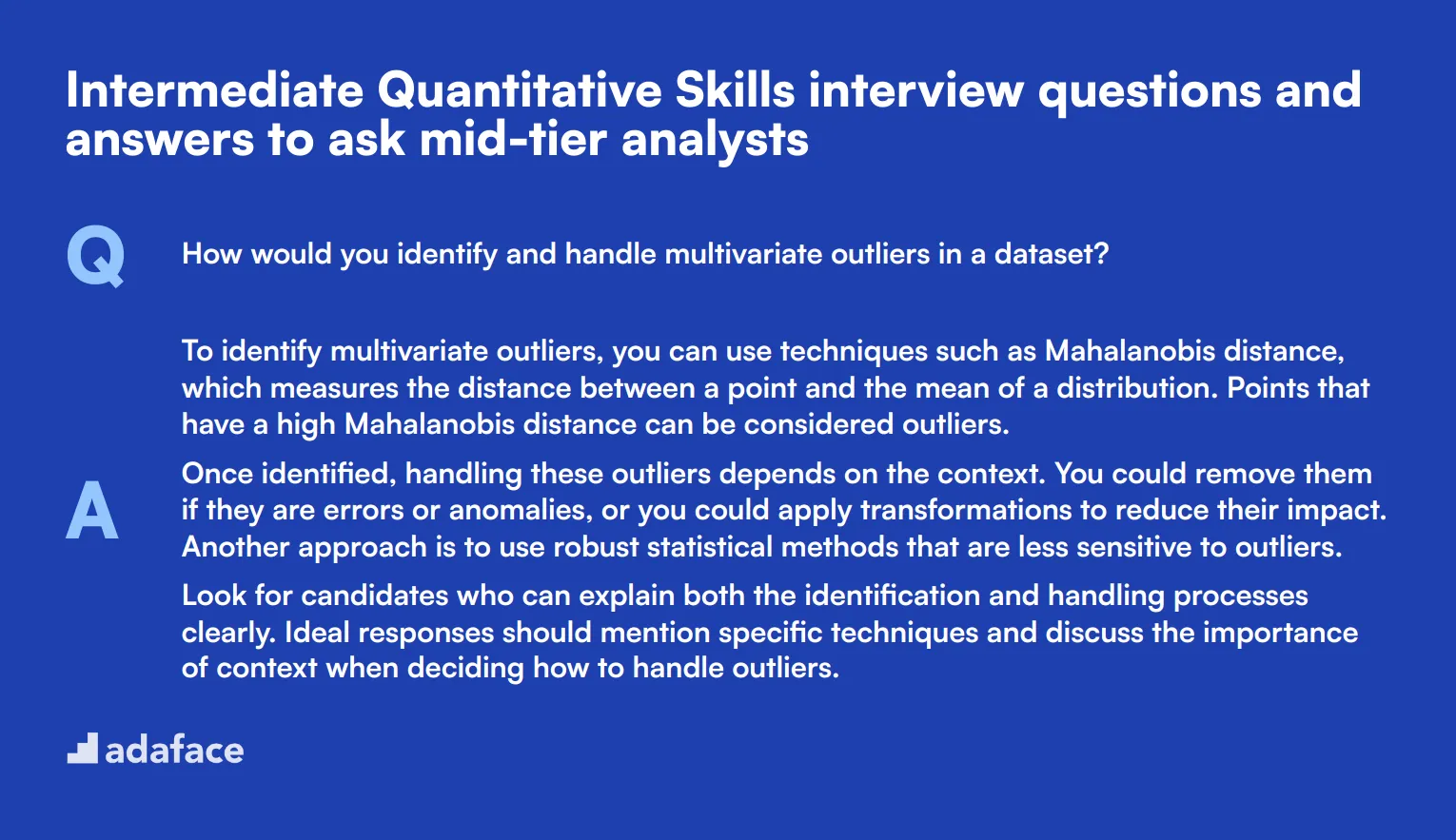
When it comes to evaluating mid-tier analysts, you need questions that go beyond the basics but aren't overly technical. This list of intermediate quantitative skills interview questions will help you find candidates who can tackle real-world data challenges with confidence and clarity.
1. How would you identify and handle multivariate outliers in a dataset?
To identify multivariate outliers, you can use techniques such as Mahalanobis distance, which measures the distance between a point and the mean of a distribution. Points that have a high Mahalanobis distance can be considered outliers.
Once identified, handling these outliers depends on the context. You could remove them if they are errors or anomalies, or you could apply transformations to reduce their impact. Another approach is to use robust statistical methods that are less sensitive to outliers.
Look for candidates who can explain both the identification and handling processes clearly. Ideal responses should mention specific techniques and discuss the importance of context when deciding how to handle outliers.
2. Can you describe a time when you had to present complex data findings to a non-technical audience? How did you ensure they understood?
When presenting complex data findings to a non-technical audience, I focus on simplifying the message without losing the essence of the data. I usually start with the main insights and then use visual aids like charts and graphs to illustrate these points.
I also use analogies and real-world examples to relate the data to something they are familiar with. For instance, comparing data trends to everyday phenomena can make the information more relatable and easier to understand.
Recruiters should look for candidates who can clearly articulate their approach to simplifying complex data. An ideal answer will show the candidate's ability to adapt their communication style to different audiences.
3. How do you approach validating the results of a predictive model?
To validate the results of a predictive model, I start with a holdout dataset that was not used during model training. This helps to test the model's ability to generalize to new data. Common techniques include cross-validation, where the data is split into multiple subsets to train and test the model multiple times.
I also look at performance metrics such as accuracy, precision, recall, and F1 score, depending on the problem type. Additionally, I check for overfitting by comparing the model's performance on the training data versus the validation data.
An ideal candidate should mention multiple validation techniques and explain why they are important. Look for a thorough understanding of performance metrics and the ability to detect overfitting.
4. What steps would you take to ensure the accuracy and reliability of your data analysis?
Ensuring the accuracy and reliability of data analysis involves several steps. First, I start with data cleaning, which includes handling missing values, correcting errors, and removing duplicates. I also perform exploratory data analysis (EDA) to understand the data's structure and detect anomalies.
Next, I ensure the analysis is reproducible by documenting my code and methodology. I also use version control systems to track changes. Finally, I validate my findings by cross-checking with multiple data sources or using different analytical methods.
Candidates should demonstrate a systematic approach to data analysis. Look for mentions of specific techniques and tools used for data cleaning, EDA, and validation. The focus should be on meticulousness and attention to detail.
5. How do you decide which data visualization technique to use for a particular dataset?
Choosing the right data visualization technique depends on the nature of the data and the message you want to convey. For categorical data, I might use bar charts or pie charts. For continuous data, line charts or histograms are more appropriate.
I also consider the audience and the context. For example, a heatmap might be useful to show correlations in a more technical setting, while a simple bar chart could be better for a general audience. The goal is to choose a visualization that makes the data easily understandable and highlights the key insights.
Recruiters should look for candidates who can justify their choices of visualization techniques. An ideal response will demonstrate an understanding of different types of data and their appropriate visual representations.
6. Explain how you would perform a root cause analysis for an unexpected drop in sales.
To perform a root cause analysis for a drop in sales, I would start by gathering and examining all relevant data, such as sales figures, customer feedback, market trends, and any recent changes in marketing strategies or product offerings.
I would then use techniques like the 5 Whys or Fishbone Diagram to systematically investigate the potential causes. This involves asking 'why' repeatedly until the underlying issue is identified. I might also perform a regression analysis to see if any variables are significantly impacting sales.
Candidates should demonstrate a methodical approach to root cause analysis. Look for mentions of specific techniques and a clear explanation of how they would gather and analyze data to identify the root cause.
7. How do you stay updated with the latest trends and technologies in data analysis?
I stay updated with the latest trends and technologies in data analysis by regularly reading industry blogs, research papers, and following thought leaders on platforms like LinkedIn and Twitter. I also attend webinars, conferences, and online courses to keep my skills sharp.
Participating in professional communities and forums, such as those on Reddit or specialized data analyst job descriptions, helps me stay informed about new tools and techniques. Continuous learning is key in a fast-evolving field like data analysis.
An ideal candidate should show a proactive approach to learning and staying updated. Look for mentions of specific resources, communities, or courses they follow to keep up with industry developments.
8. What methods do you use to ensure that your data modeling assumptions are valid?
To ensure that my data modeling assumptions are valid, I start by conducting exploratory data analysis (EDA) to understand the data's distribution and relationships. I also perform statistical tests to check for assumptions like normality, linearity, and homoscedasticity.
I use diagnostic plots, such as residual plots, to visually inspect the assumptions. Additionally, I might perform sensitivity analysis to see how changes in assumptions affect the model's results. If assumptions are violated, I consider transforming the data or using alternative modeling techniques.
Candidates should demonstrate a thorough understanding of the importance of validating modeling assumptions. Look for specific methods and tests they use, as well as their approach to handling violations of these assumptions.
9. How do you approach integrating data from multiple sources into a single analysis?
Integrating data from multiple sources involves several steps. First, I ensure that the data is compatible in terms of format and structure. This often involves cleaning and transforming the data to ensure consistency.
I then use techniques like merging and joining datasets based on common keys or identifiers. I also pay close attention to data quality issues, such as duplicates or mismatched records, and resolve them accordingly. Finally, I validate the integrated dataset by cross-checking key metrics or running sanity checks.
Look for candidates who can clearly explain the process of integrating data. An ideal answer should mention specific techniques and tools they use, as well as the importance of data quality and validation.
10. Can you explain how you would use clustering techniques in data analysis?
Clustering techniques are used to group similar data points together based on their characteristics. One common method is K-means clustering, where data points are assigned to clusters based on their distance to the nearest cluster center. Another method is hierarchical clustering, which builds a tree-like structure of nested clusters.
Clustering can be useful for customer segmentation, identifying patterns, or anomaly detection. For example, in customer segmentation, clustering can help identify distinct groups of customers with similar behaviors, which can then inform targeted marketing strategies.
Candidates should show an understanding of different clustering techniques and their applications. Look for examples of how they have used clustering in past projects and their ability to explain the benefits of clustering in data analysis.
15 advanced Quantitative Skills interview questions to ask senior analysts
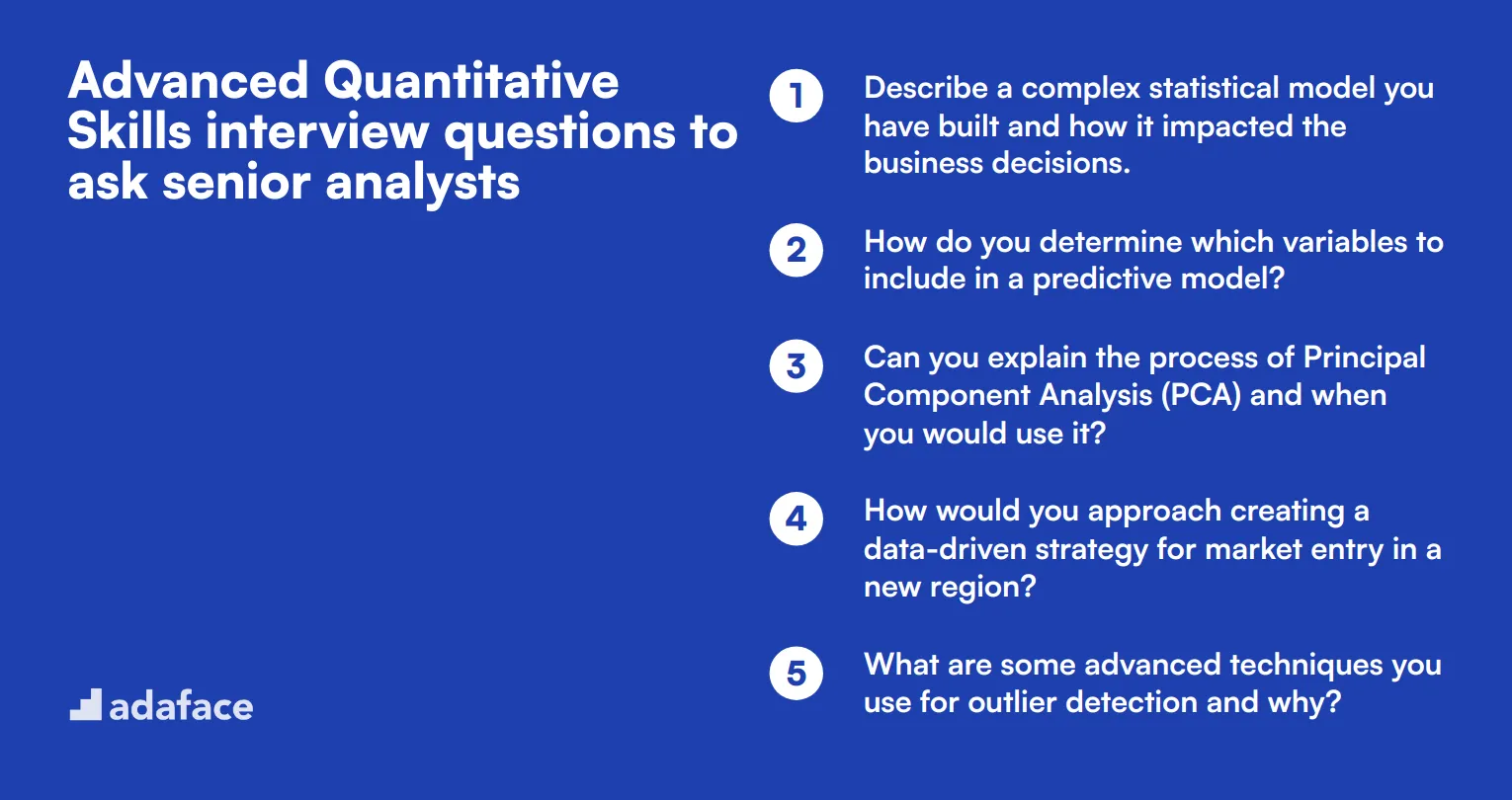
To ensure that your senior analysts possess advanced quantitative skills, it's essential to ask targeted questions during interviews. Use these questions to gauge candidates' proficiency and their ability to handle complex analytical tasks, as outlined in our data analyst job description.
- Describe a complex statistical model you have built and how it impacted the business decisions.
- How do you determine which variables to include in a predictive model?
- Can you explain the process of Principal Component Analysis (PCA) and when you would use it?
- How would you approach creating a data-driven strategy for market entry in a new region?
- What are some advanced techniques you use for outlier detection and why?
- Explain how you would validate the assumptions of a linear regression model.
- Can you discuss a time when you had to use advanced SQL queries to solve a business problem?
- How do you handle the challenge of integrating unstructured data into your analysis?
- Explain your experience with any advanced data visualization tools and how you use them to communicate insights.
- Describe a scenario where you automated a data analysis process. What tools and techniques did you use?
- How do you perform hypothesis testing when comparing multiple groups?
- Explain how you would conduct a rolling forecast and its advantages in financial planning.
- Can you provide an example of a data science project where you employed machine learning algorithms?
- Describe your approach to feature engineering and why it's crucial for model performance.
- How do you ensure the reproducibility of your analysis and results?
9 Quantitative Skills interview questions and answers related to statistical models
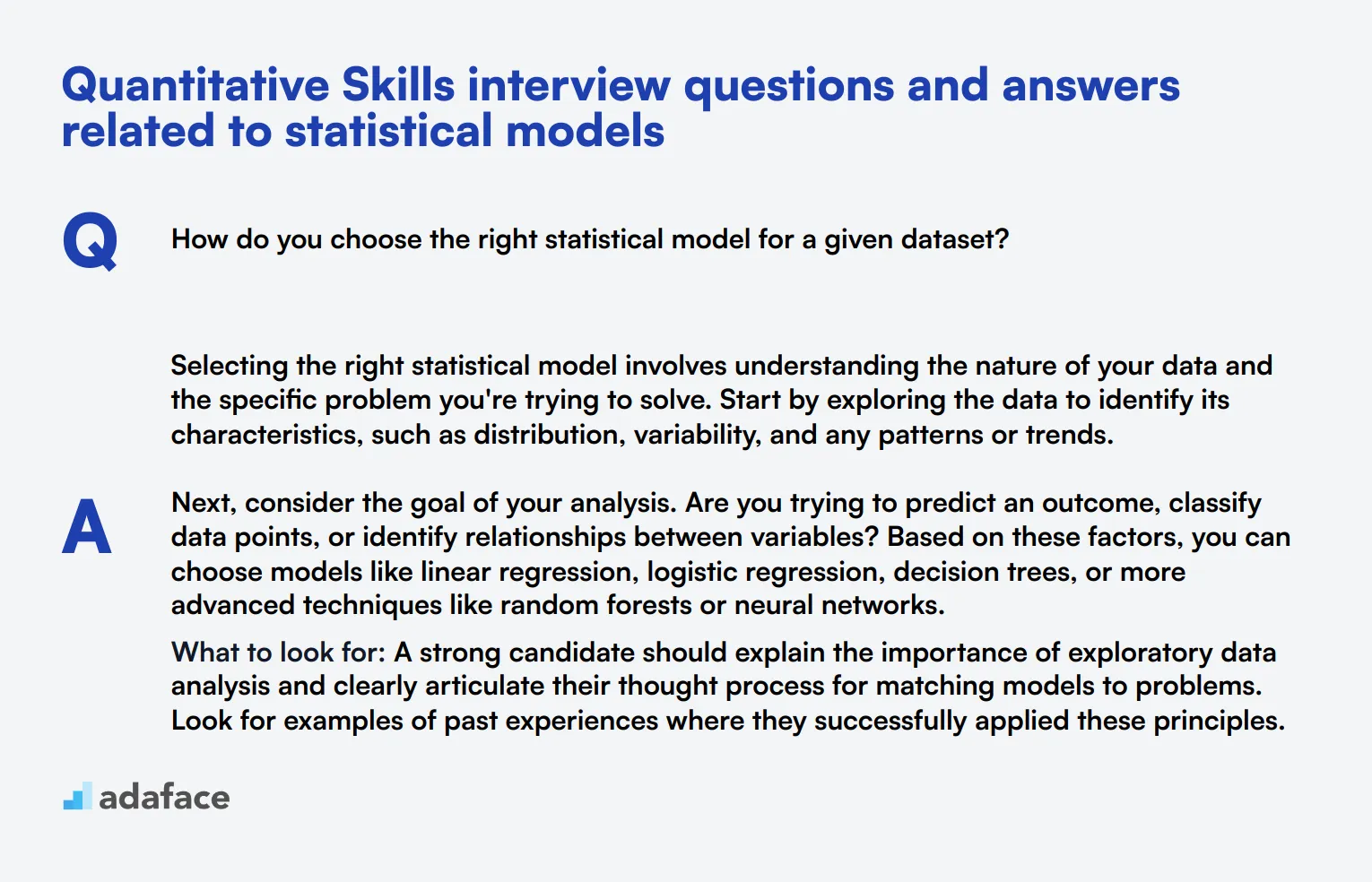
If you want to gauge a candidate's grasp on statistical models, these questions will help you do just that. Use this list when you're interviewing for roles that require strong quantitative skills and a solid understanding of statistical concepts.
1. How do you choose the right statistical model for a given dataset?
Selecting the right statistical model involves understanding the nature of your data and the specific problem you're trying to solve. Start by exploring the data to identify its characteristics, such as distribution, variability, and any patterns or trends.
Next, consider the goal of your analysis. Are you trying to predict an outcome, classify data points, or identify relationships between variables? Based on these factors, you can choose models like linear regression, logistic regression, decision trees, or more advanced techniques like random forests or neural networks.
What to look for: A strong candidate should explain the importance of exploratory data analysis and clearly articulate their thought process for matching models to problems. Look for examples of past experiences where they successfully applied these principles.
2. Explain how you would validate a statistical model.
Model validation is essential to ensure your model's reliability and accuracy. One common approach is to split your dataset into a training set and a test set. The model is trained on the training set and then evaluated on the test set to assess its performance.
Other techniques include cross-validation, where the data is divided into multiple subsets, and the model is trained and tested on different combinations of these subsets. This helps in ensuring the model generalizes well to unseen data.
What to look for: Ideal candidates should mention both the importance of splitting data and the use of cross-validation techniques. They should also discuss metrics they use to evaluate model performance, such as accuracy, precision, recall, or F1 score.
3. How do you handle multicollinearity in a dataset?
Multicollinearity occurs when independent variables in a regression model are highly correlated, which can distort the results. To address this, one approach is to remove or combine highly correlated variables to reduce redundancy.
Another method is to use regularization techniques like Ridge or Lasso regression, which add a penalty for higher coefficients in the model, thus reducing the impact of multicollinearity.
What to look for: Look for candidates who can explain these techniques clearly and provide examples of when and how they've dealt with multicollinearity in past projects. Their ability to articulate the impact of multicollinearity on model interpretation is crucial.
4. Describe how you would perform feature selection for a statistical model.
Feature selection involves choosing the most relevant variables for your model to improve its performance and simplify interpretation. Techniques include removing features with low variance, using correlation matrices, and employing methods like forward selection, backward elimination, or recursive feature elimination.
Advanced methods involve using model-based techniques like feature importance from tree-based models or regularization methods that shrink less important feature coefficients to zero.
What to look for: A strong candidate should understand and explain various feature selection techniques and provide examples of their practical application. They should also discuss the impact of feature selection on model performance and interpretability.
5. How do you address overfitting in a statistical model?
Overfitting occurs when a model learns noise in the training data, performing well on training data but poorly on unseen data. Strategies to prevent overfitting include using simpler models, applying cross-validation, and reducing the number of features.
Regularization techniques like Ridge and Lasso regression can also help by adding a penalty to large coefficients, thus discouraging overfitting. Pruning techniques in decision trees and dropout in neural networks are other effective methods.
What to look for: Candidates should clearly explain these strategies and provide examples of how they've successfully reduced overfitting in past projects. Look for their understanding of the balance between model complexity and generalization.
6. Explain the concept of a confusion matrix and how you would use it.
A confusion matrix is a table used to evaluate the performance of a classification model. It shows the actual vs. predicted classifications, allowing you to see true positives, true negatives, false positives, and false negatives.
By analyzing the confusion matrix, you can calculate important metrics like accuracy, precision, recall, and the F1 score, which provide insights into the model's performance and areas for improvement.
What to look for: Look for candidates who can clearly explain how to interpret a confusion matrix and calculate related metrics. Their ability to discuss the implications of these metrics on model performance is essential.
7. How would you handle missing data in a dataset?
Handling missing data is crucial for ensuring the accuracy of your analysis. Common methods include imputation (filling in missing values with the mean, median, or mode) and using algorithms that can handle missing data directly.
More advanced techniques involve using models to predict missing values based on other variables or using data augmentation methods to create synthetic data points.
What to look for: Strong candidates should understand various imputation techniques and discuss the pros and cons of each. Look for their ability to choose the appropriate method based on the context and explain their rationale.
8. Can you explain the concept of bias-variance tradeoff?
The bias-variance tradeoff is a fundamental concept in machine learning and statistics. Bias refers to errors introduced by approximating a real-world problem with a simplified model, while variance refers to errors introduced by the model's sensitivity to small fluctuations in the training data.
A good model strikes a balance between bias and variance, ensuring it generalizes well to new data. High bias results in underfitting, and high variance results in overfitting. Techniques like cross-validation and regularization can help manage this tradeoff.
What to look for: Candidates should demonstrate a clear understanding of the bias-variance tradeoff and provide examples of how they've managed it in their work. Their ability to articulate the impact on model performance is key.
9. How do you interpret the coefficients of a linear regression model?
In a linear regression model, the coefficients represent the change in the dependent variable for a one-unit change in the independent variable, holding all other variables constant. They provide insights into the strength and direction of relationships between variables.
It's important to check the significance of these coefficients using p-values and confidence intervals to ensure they are meaningful. Additionally, examining the residuals helps assess the adequacy of the model.
What to look for: Look for candidates who can clearly explain the interpretation of coefficients and discuss the importance of significance testing. Their understanding of residual analysis and model diagnostics is crucial for a thorough response.
Which Quantitative Skills skills should you evaluate during the interview phase?
While a single interview session may not reveal everything about a candidate's capabilities, focusing on core quantitative skills can provide significant insights into their potential performance. Especially in analytical roles, these skills are indicative of a candidate's ability to handle complex data-driven tasks effectively.
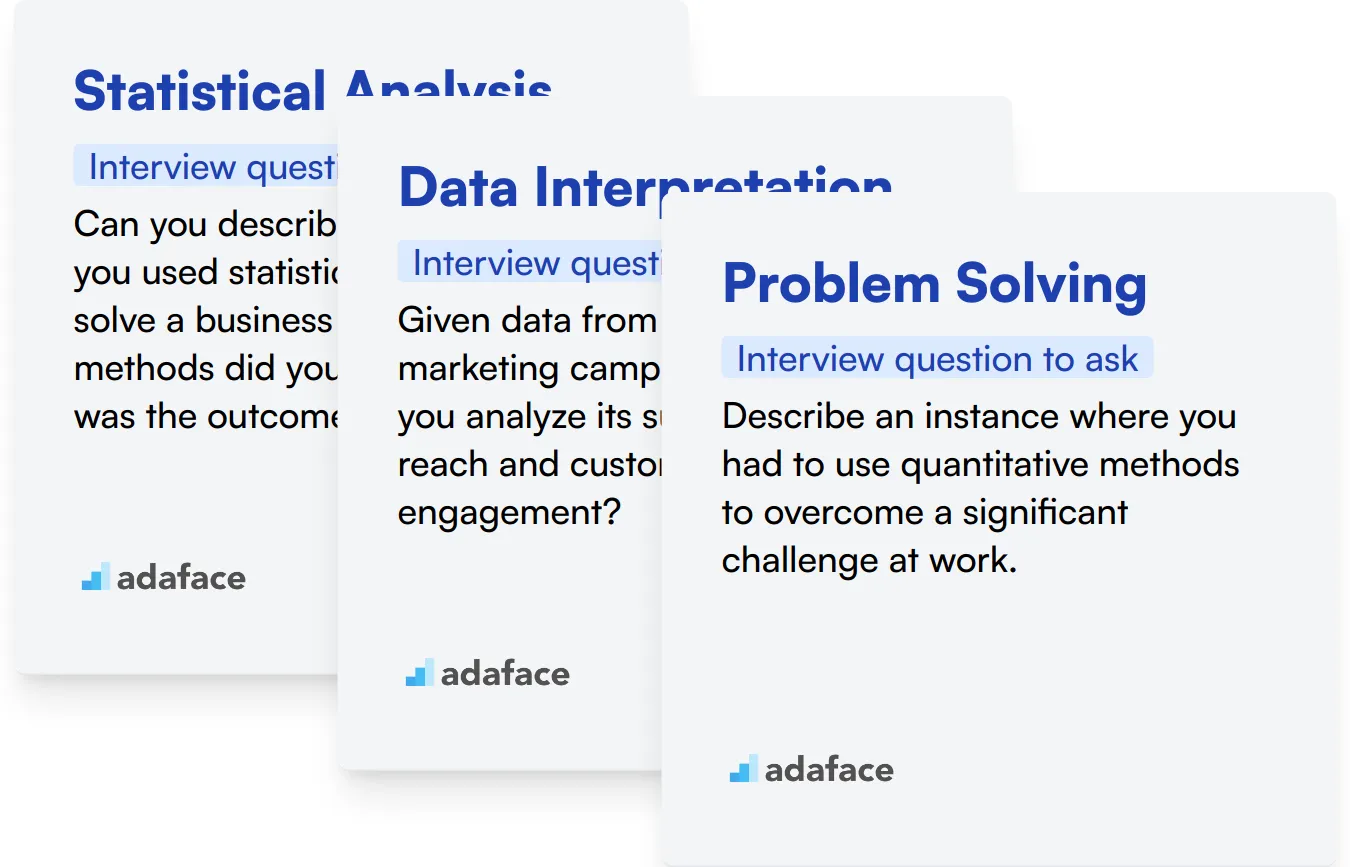
Statistical Analysis
Statistical analysis is the backbone of quantitative reasoning, enabling analysts to interpret data, recognize patterns and make predictions. Mastery of this skill is necessary for making informed decisions based on data.
To assess candidates' proficiency in statistical analysis, consider using a targeted assessment like the Statistical Analysis Test which includes relevant multiple-choice questions to filter out candidates effectively.
During the interview, it's also beneficial to ask specific questions that evaluate their practical application of statistical theories.
Can you describe a time when you used statistical analysis to solve a business problem? What methods did you use and what was the outcome?
Look for detailed explanations that highlight the candidate's approach to problem-solving and their ability to translate data into actionable insights.
Data Interpretation
Data interpretation skills are critical for understanding raw data's real-world implications, allowing analysts to transform numbers into strategic insights.
To effectively measure data interpretation skills, you could leverage an assessment such as the Data Interpretation Test, which challenges candidates with practical data scenarios.
Incorporate interview questions that push the candidate to demonstrate their data interpretation capabilities.
Given data from a recent marketing campaign, how would you analyze its success in terms of reach and customer engagement?
The answer should indicate the candidate's ability to derive meaningful conclusions from marketing data and suggest improvements or strategies based on those insights.
Problem Solving
Problem-solving is crucial for quantitative roles as it directly influences an analyst's ability to propose viable solutions under constraints based on quantitative analysis.
A problem-solving skill test can be an effective tool to assess this ability. While Adaface does not have a specific test titled 'Problem Solving', you can explore tests like the technical aptitude test that cover similar competencies.
Tailor your interview questions to evaluate how candidates approach complex, unforeseen challenges.
Describe an instance where you had to use quantitative methods to overcome a significant challenge at work.
Focus on understanding the logical sequence of their thought process and their effectiveness in applying quantitative methods to solve real-world problems.
Optimize Your Hiring Process with Quantitative Skills Tests from Adaface
If you're aiming to hire professionals with precise quantitative skills, verifying these skills is a crucial first step. Making sure candidates possess the necessary capabilities ensures a good fit for your team.
Using skill tests is the most direct way to assess these capabilities. We recommend checking out the Quantitative Aptitude Test or the Data Interpretation Test available in our test library for accurate assessment.
After utilizing these tests, you can efficiently filter and shortlist the top candidates. This streamlined process allows you to invite only the most promising applicants for interviews, saving time and resources.
Ready to take the next step? Sign up on our platform through this link and explore the various assessments we offer on our online assessment platform.
Quantitative Aptitude Online Test
Download Quantitative Skills interview questions template in multiple formats
Quantitative Skills Interview Questions FAQs
Common questions include problem-solving scenarios, statistical model analysis, and questions on data interpretation skills.
Focus on basic problem-solving, data analysis tasks, and understanding of fundamental statistical concepts.
For senior analysts, ask advanced questions on statistical models, complex data scenarios, and experience-based problem-solving.
They help gauge a candidate's ability to handle data, perform analysis, and derive insights which are crucial for many roles.
Adaface offers tailored quantitative skills tests to streamline your hiring process and evaluate candidates effectively.
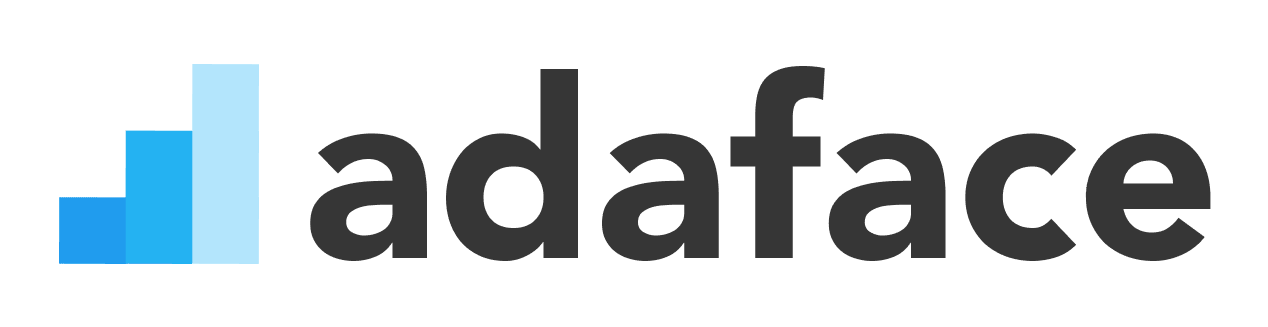
40 min skill tests.
No trick questions.
Accurate shortlisting.
We make it easy for you to find the best candidates in your pipeline with a 40 min skills test.
Try for freeRelated posts
Free resources
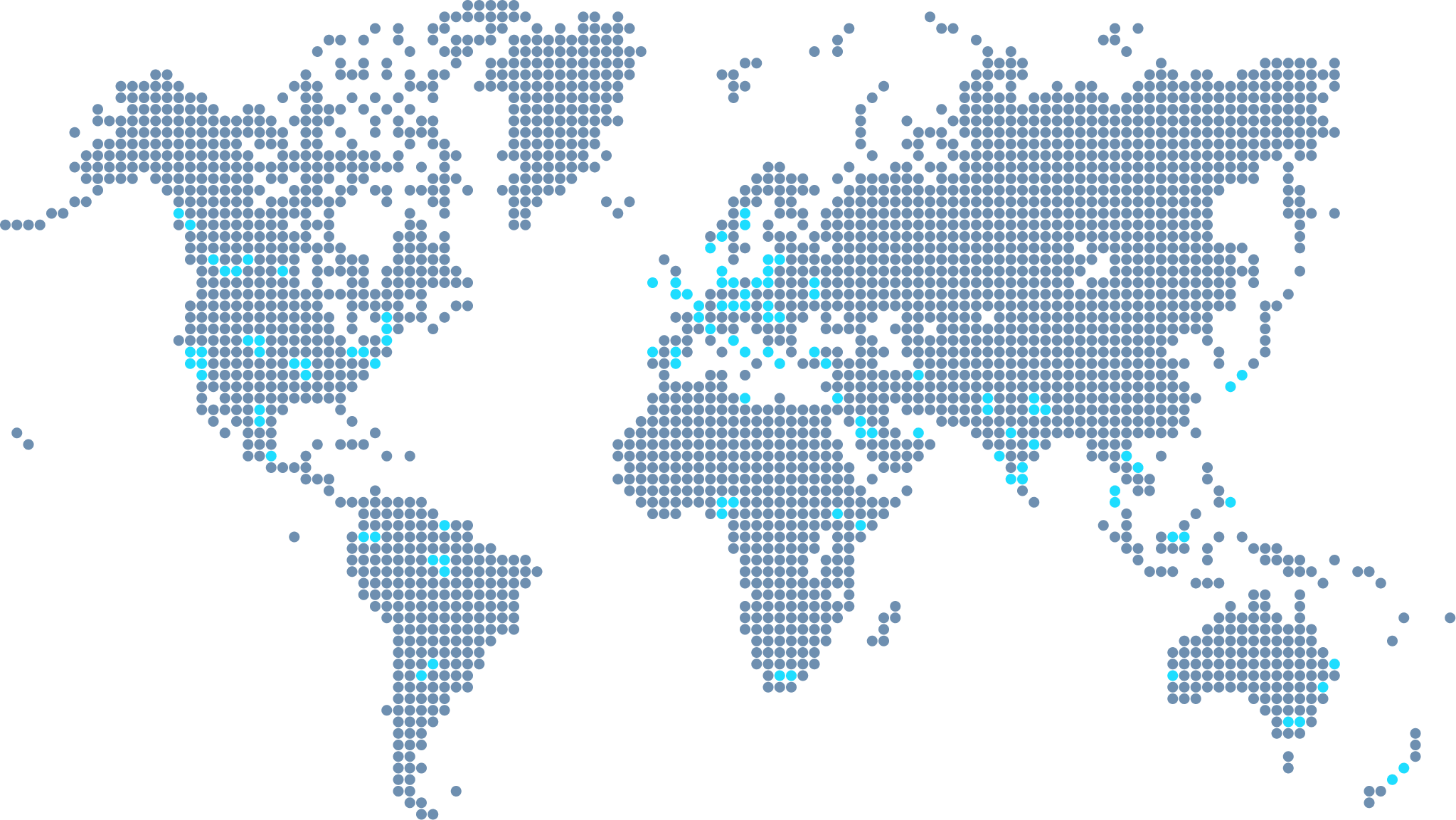
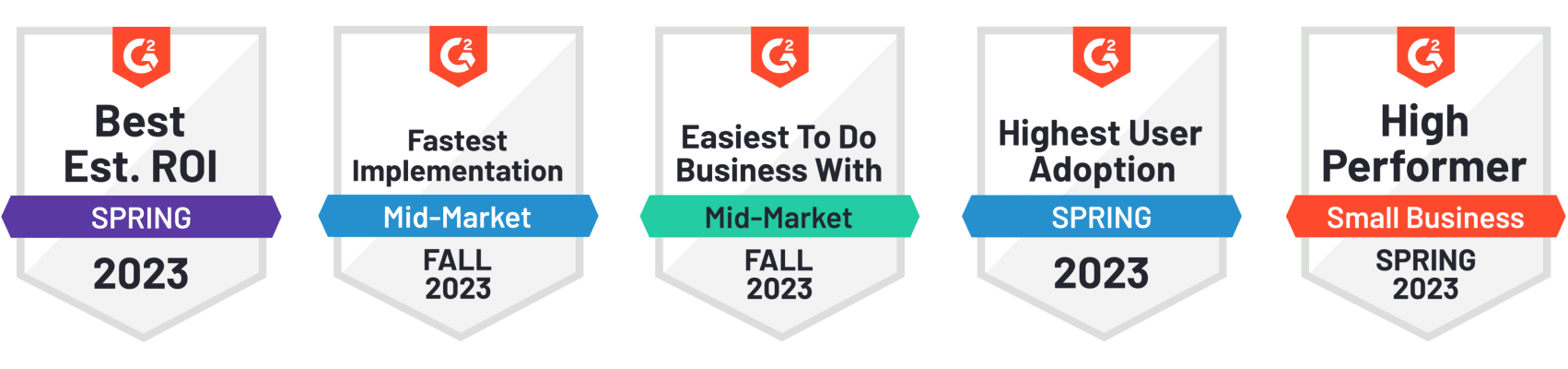