Data interpretation skills are crucial for analysts to extract meaningful insights from complex information. Effective data interpretation interview questions help recruiters and hiring managers identify candidates who can analyze, visualize, and communicate data-driven insights accurately.
This blog post provides a comprehensive list of data interpretation interview questions for various skill levels and roles. From basic questions for junior analysts to advanced scenarios for top-tier professionals, we cover a wide range of topics including data analysis techniques and visualization methods.
By using these questions, you can effectively assess candidates' ability to interpret data and make informed decisions. Consider combining these interview questions with a pre-employment data interpretation assessment for a more thorough evaluation of candidates' skills.
Table of contents
10 basic Data Interpretation interview questions and answers to assess candidates
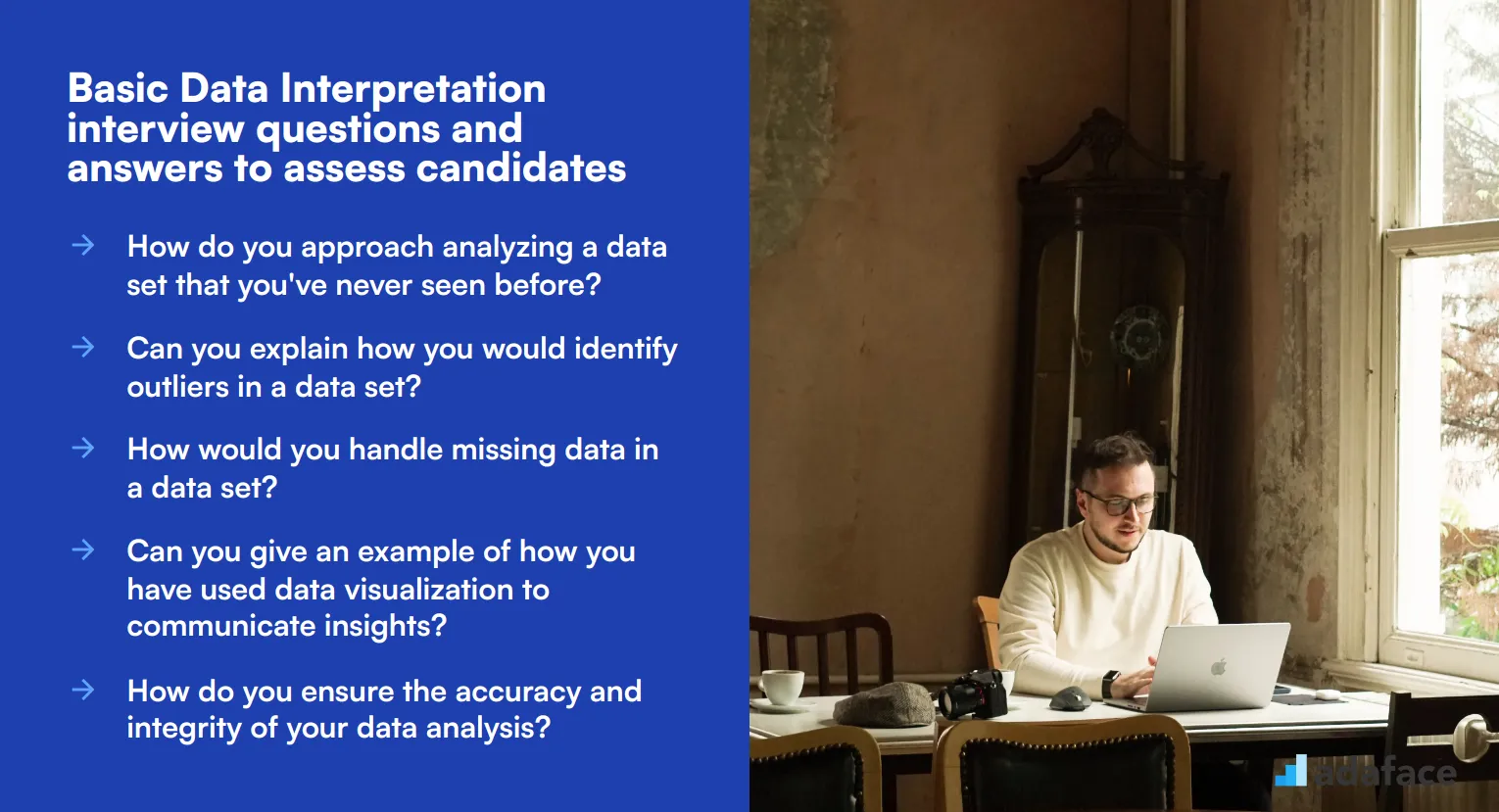
Looking to evaluate how well candidates can interpret and analyze data? These 10 basic Data Interpretation interview questions will help you assess their skills and fit for the role. Use them to gain insights into their problem-solving abilities, attention to detail, and analytical thinking.
1. How do you approach analyzing a data set that you've never seen before?
When analyzing an unfamiliar data set, I start by understanding the context and the goals of the analysis. I review any relevant documentation and data dictionaries to understand the variables and their relationships. Then, I perform a preliminary analysis to get a sense of the data's structure and quality.
Next, I clean the data by handling missing values, outliers, and inconsistencies. This includes normalizing data formats and removing duplicates. Once the data is clean, I use exploratory data analysis (EDA) techniques to identify patterns, trends, and anomalies. This helps me formulate hypotheses and insights.
An ideal candidate will demonstrate a methodical approach to data analysis, show an understanding of data cleaning processes, and be familiar with EDA techniques. Look for candidates who can articulate their process clearly and provide specific examples from past experiences.
2. Can you explain how you would identify outliers in a data set?
To identify outliers, I start with a visual inspection using plots like box plots and scatter plots, which can highlight data points that deviate significantly from the rest. I also calculate statistical measures such as the interquartile range (IQR) and standard deviation. Data points that fall beyond 1.5 times the IQR or more than three standard deviations from the mean are typically considered outliers.
In addition to visual and statistical methods, I consider the context of the data. Sometimes, outliers can provide valuable insights rather than being just anomalies. For example, in sales data, outliers might indicate a high-performing product or an error in data entry.
Candidates should be able to explain both visual and statistical methods for identifying outliers and discuss the importance of context in interpreting these anomalies. Follow up by asking for specific examples of how they have handled outliers in their previous work.
3. How would you handle missing data in a data set?
Handling missing data depends on the nature and extent of the missing values. If the amount of missing data is small, I might use imputation techniques such as mean, median, or mode substitution. For more significant gaps, I might use more advanced methods like regression imputation or multiple imputation.
Another approach is to analyze the pattern of missingness. If data is missing completely at random, simpler methods might suffice. If not, more sophisticated techniques may be needed to avoid bias. In some cases, it might be appropriate to remove the affected records or variables, especially if they are not critical to the analysis.
An ideal candidate will show an understanding of different imputation techniques and the importance of analyzing the pattern of missing data. Look for candidates who can explain their thought process and justify their chosen methods based on the context.
4. Can you give an example of how you have used data visualization to communicate insights?
In my previous role, I used data visualization to present the results of a sales performance analysis. I created a series of interactive dashboards using tools like Tableau and Power BI to illustrate key metrics such as sales trends, regional performance, and product categories. These visualizations helped stakeholders quickly grasp complex data insights and make informed decisions.
One specific example involved a heatmap to show sales performance across different regions. The heatmap highlighted areas with high and low sales, making it easy for the management team to identify regions needing attention. I also used line charts to show sales trends over time, which helped in forecasting future sales.
Candidates should be able to provide specific examples of how they have used data visualization to communicate insights effectively. Look for their ability to choose the right visualization tools and techniques to make data accessible and actionable for stakeholders.
5. How do you ensure the accuracy and integrity of your data analysis?
Ensuring accuracy and integrity starts with a thorough data cleaning process, which involves checking for and correcting errors, handling missing data, and validating data against known benchmarks. I also ensure that the data sources are reliable and consistent.
I use validation techniques such as cross-checking results with different methods, conducting sanity checks, and having peer reviews of my analysis. Documenting the data cleaning and analysis steps also adds transparency and facilitates reproducibility.
Look for candidates who emphasize the importance of data cleaning, validation, and documentation. An ideal candidate will demonstrate a rigorous approach to ensuring data accuracy and integrity, with examples from their past work.
6. Can you explain a time when you had to interpret complex data to provide actionable insights?
In one project, I analyzed customer behavior data to identify factors that influenced customer churn. I started by cleaning and preparing the data, followed by exploratory data analysis to identify trends and patterns. Using logistic regression, I found that factors like customer service interactions and usage frequency were significant predictors of churn.
I presented the findings to the management team using clear visualizations and recommended strategies to improve customer retention, such as enhancing customer service and increasing engagement through targeted campaigns. These insights led to a 15% reduction in churn over the next quarter.
Candidates should be able to provide specific examples of how they have turned complex data into actionable insights. Look for their ability to explain both the technical and business aspects of their analysis and the impact of their recommendations.
7. What methods do you use to validate your data analysis results?
To validate my data analysis results, I use several methods. First, I cross-verify results using different analytical techniques to ensure consistency. For example, if conducting a regression analysis, I might also use decision trees to see if similar patterns emerge.
I also perform sensitivity analysis to understand how changes in the data or assumptions affect the outcomes. Peer reviews and stakeholder feedback are essential components of my validation process, as they provide additional perspectives and help identify any overlooked issues.
An ideal candidate will emphasize the importance of cross-verification, sensitivity analysis, and peer reviews in validating their results. Look for a thorough and systematic approach to ensure the reliability of their analysis.
8. How do you prioritize which data insights to act on first?
Prioritizing data insights involves assessing the potential impact and feasibility of each insight. I evaluate insights based on their alignment with business objectives, the resources required to act on them, and the expected return on investment. High-impact, low-effort insights are typically prioritized first.
I also consider the urgency of the insights. For example, if an insight reveals a critical issue that needs immediate attention, it will be prioritized over less urgent findings. Collaborating with stakeholders helps ensure that the priorities align with the broader business strategy.
Candidates should be able to explain their criteria for prioritizing insights and demonstrate an understanding of balancing impact, feasibility, and urgency. Look for their ability to align their recommendations with business goals.
9. Describe a situation where you had to present your data findings to a non-technical audience.
In a previous role, I had to present the results of a market analysis to a team of marketing professionals. I focused on using clear, simple language and avoided technical jargon. Visual aids like charts and graphs helped convey complex data in an accessible way.
I also used storytelling techniques to build a narrative around the data, highlighting key insights and their implications for the marketing strategy. This approach helped the team understand the findings and take actionable steps based on the insights.
Look for candidates who can demonstrate their ability to communicate complex data findings to non-technical audiences effectively. An ideal candidate will use clear language, visual aids, and storytelling techniques to make their insights accessible and engaging.
10. How do you stay updated with the latest trends and tools in data analysis?
I stay updated by regularly reading industry blogs, following thought leaders on social media, and participating in online forums and communities. I also attend webinars, workshops, and conferences to learn about the latest trends and tools in data analysis.
Continuous learning is essential, so I take online courses and certifications to enhance my skills. Subscribing to newsletters and joining professional organizations also helps me stay informed about new developments and best practices in the field.
Candidates should show a commitment to continuous learning and staying current with industry trends. Look for their engagement with professional communities and their proactive approach to skill development.
20 Data Interpretation interview questions to ask junior analysts
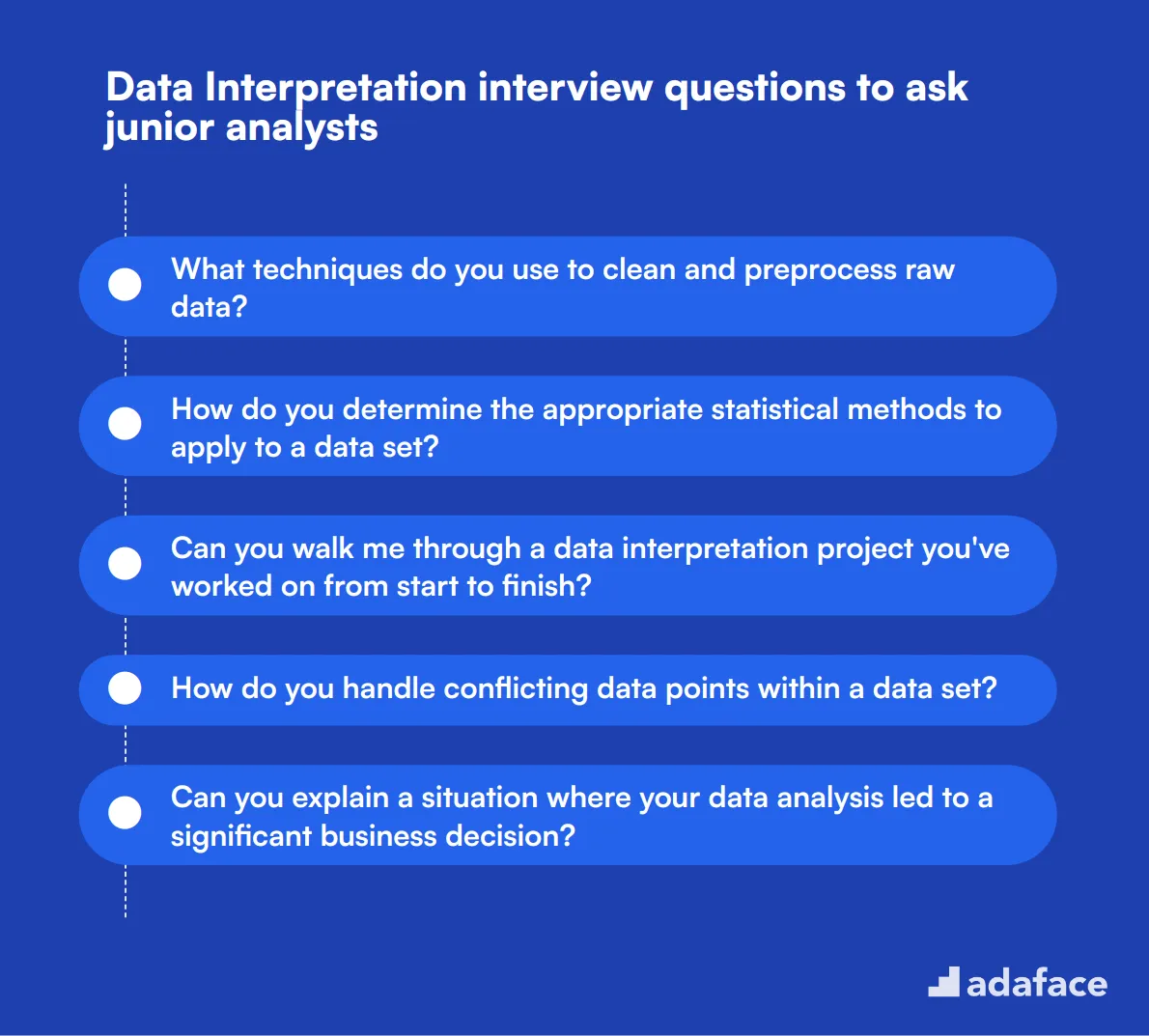
To determine whether junior analysts possess the right skills for your team, ask them some of these data interpretation interview questions. This list will help you gauge their analytical abilities, problem-solving approach, and how they communicate insights. For more information on what skills are essential for different roles, check out this data analyst job description.
- What techniques do you use to clean and preprocess raw data?
- How do you determine the appropriate statistical methods to apply to a data set?
- Can you walk me through a data interpretation project you've worked on from start to finish?
- How do you handle conflicting data points within a data set?
- Can you explain a situation where your data analysis led to a significant business decision?
- What tools do you prefer for data analysis and why?
- How do you ensure that your data interpretations are free from bias?
- Describe your experience with data mining techniques.
- How do you approach performing a trend analysis on historical data?
- Can you discuss a time when you had to debug a problematic data set?
- How do you measure the success of your data interpretation efforts?
- What steps do you take to validate the sources of your data?
- How do you communicate your findings when there is uncertainty in the data?
- What is your experience with A/B testing and how do you interpret the results?
- How do you balance the need for thorough analysis with tight deadlines?
- Can you explain a complex data concept to someone without a data background?
- What challenges have you faced when collaborating with other team members on data projects?
- How do you keep track of the various assumptions you make during your analysis?
- What role does domain knowledge play in your data interpretation process?
- How do you handle large datasets that your tools might struggle to process?
10 intermediate Data Interpretation interview questions and answers to ask mid-tier analysts.
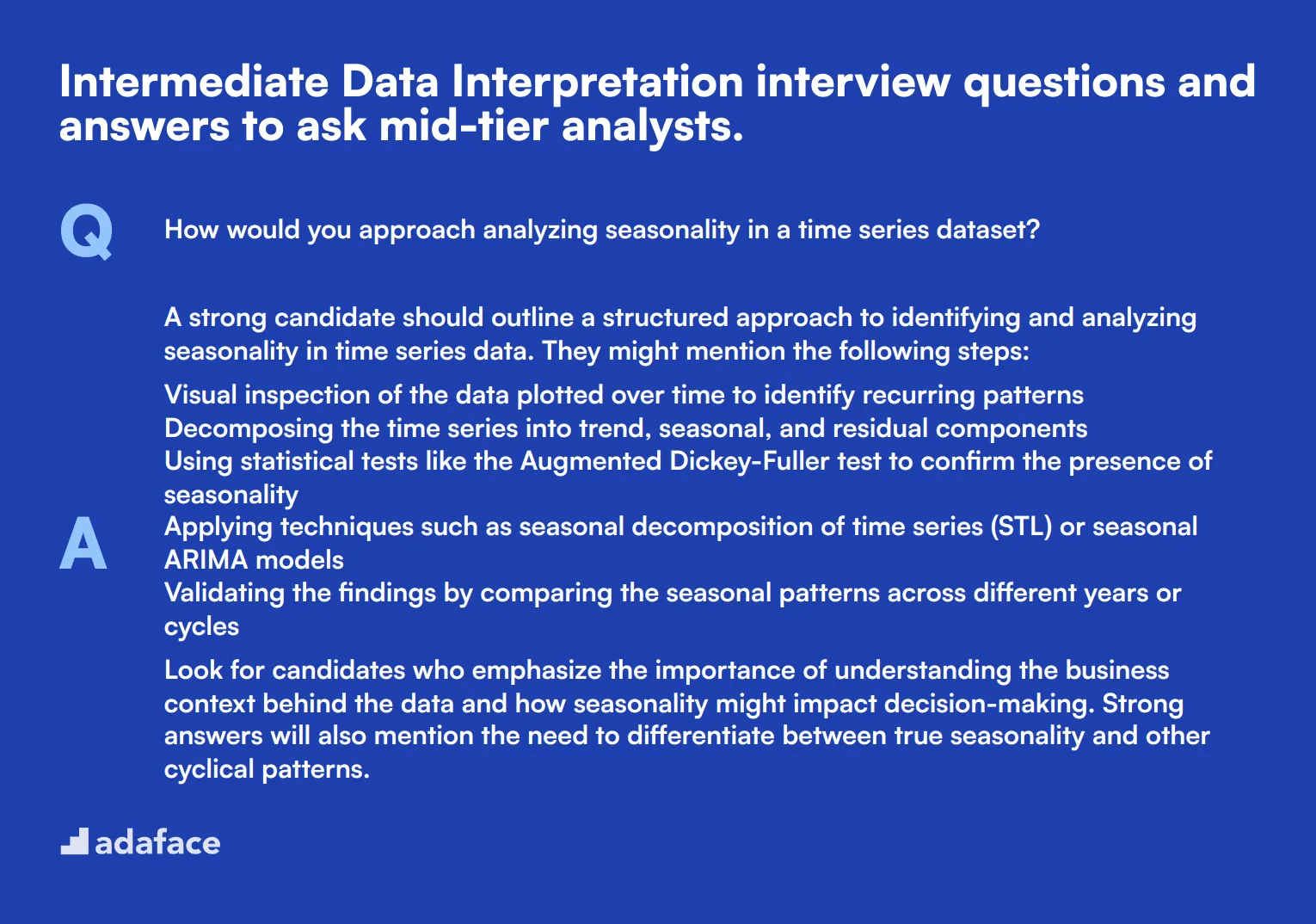
Ready to level up your data interpretation interviews? These 10 intermediate questions are perfect for assessing mid-tier analysts. They'll help you gauge a candidate's ability to dive deeper into data, spot trends, and communicate insights effectively. Use these questions to uncover the skills that separate good analysts from great ones.
1. How would you approach analyzing seasonality in a time series dataset?
A strong candidate should outline a structured approach to identifying and analyzing seasonality in time series data. They might mention the following steps:
- Visual inspection of the data plotted over time to identify recurring patterns
- Decomposing the time series into trend, seasonal, and residual components
- Using statistical tests like the Augmented Dickey-Fuller test to confirm the presence of seasonality
- Applying techniques such as seasonal decomposition of time series (STL) or seasonal ARIMA models
- Validating the findings by comparing the seasonal patterns across different years or cycles
Look for candidates who emphasize the importance of understanding the business context behind the data and how seasonality might impact decision-making. Strong answers will also mention the need to differentiate between true seasonality and other cyclical patterns.
2. Describe a situation where correlation did not imply causation in your data analysis. How did you handle it?
An ideal response should demonstrate the candidate's critical thinking skills and ability to avoid common pitfalls in data interpretation. They might describe a scenario like:
"In a previous project, we found a strong positive correlation between ice cream sales and sunscreen purchases. Initially, it might seem that increased ice cream consumption leads to more sunscreen use. However, this is a classic case of correlation not implying causation. Both variables were actually influenced by a third factor: warm, sunny weather."
Look for candidates who explain how they communicated this nuance to stakeholders and used additional analysis techniques, such as controlled experiments or causal inference methods, to investigate true causal relationships. Strong answers will highlight the importance of considering confounding variables and the danger of making business decisions based solely on correlations.
3. How would you detect and handle multicollinearity in a regression model?
A competent analyst should be able to explain both the concept of multicollinearity and methods to address it. A good answer might include:
- Definition of multicollinearity: high correlation between independent variables in a regression model
- Detection methods:
- Correlation matrix
- Variance Inflation Factor (VIF)
- Condition number
- Handling techniques:
- Removing one of the correlated variables
- Combining correlated variables into a single feature
- Using regularization techniques like Ridge or Lasso regression
- Collecting more data or using dimensionality reduction techniques
Look for candidates who understand the implications of multicollinearity on model interpretation and prediction. They should also mention the importance of balancing statistical considerations with business context when deciding how to handle multicollinearity.
4. Explain how you would conduct a cohort analysis and what insights it can provide.
A strong response should demonstrate understanding of cohort analysis and its applications. The candidate might explain:
Cohort analysis involves grouping users based on shared characteristics or experiences within a defined time-span. Steps include:
- Defining the cohorts (e.g., customers who made their first purchase in a specific month)
- Choosing metrics to track (e.g., retention rate, average order value)
- Creating a cohort table or visualization to compare cohort performance over time
- Analyzing trends and patterns across cohorts
Look for candidates who can articulate the business intelligence insights cohort analysis can provide, such as understanding customer lifecycle, identifying factors affecting long-term customer value, and measuring the impact of changes or interventions on different user groups. Strong answers will also mention potential pitfalls, like misinterpreting results due to external factors affecting certain time periods.
5. How would you approach anomaly detection in a large dataset?
An effective answer should outline a systematic approach to identifying anomalies. A candidate might describe the following steps:
- Understand the context and define what constitutes an anomaly for the specific dataset
- Explore the data visually to spot obvious outliers
- Apply statistical methods such as Z-score, Interquartile Range (IQR), or Mahalanobis distance
- Use machine learning techniques like isolation forests, one-class SVM, or autoencoders for more complex datasets
- Validate findings and investigate root causes of identified anomalies
Look for candidates who emphasize the importance of domain knowledge in interpreting anomalies and distinguishing between true anomalies and data quality issues. Strong answers will also mention the need to balance sensitivity and specificity in anomaly detection, and discuss how to handle anomalies once identified (e.g., remove, transform, or flag for further investigation).
6. Describe a time when you had to explain a complex statistical concept to a non-technical stakeholder. How did you approach it?
This question assesses the candidate's communication skills and ability to translate technical concepts into business language. A strong answer might describe:
"I once had to explain the concept of statistical significance to a marketing manager who was skeptical about A/B test results. I used a coin-flipping analogy, explaining that if you flip a coin 10 times and get 6 heads, it doesn't necessarily mean the coin is biased. But if you flip it 1000 times and get 600 heads, you can be more confident that something is influencing the results."
Look for candidates who demonstrate creativity in using analogies or visual aids to simplify complex ideas. They should also mention how they tailored their explanation to the stakeholder's background and interests, and how they confirmed understanding through questions and feedback. Strong answers will highlight the importance of bridging the gap between technical analysis and business decision-making.
7. How would you approach feature selection for a predictive model?
A comprehensive answer should cover various feature selection techniques and their applications. The candidate might outline the following approach:
- Start with domain knowledge to identify potentially relevant features
- Use correlation analysis to understand relationships between features and the target variable
- Apply filter methods like chi-squared test or mutual information
- Utilize wrapper methods such as recursive feature elimination
- Implement embedded methods like Lasso or Random Forest feature importance
- Consider dimensionality reduction techniques like PCA if appropriate
- Validate selected features through cross-validation and model performance metrics
Look for candidates who emphasize the importance of balancing model performance with interpretability and computational efficiency. Strong answers will also mention the need to consider multicollinearity and the potential for overfitting when selecting features. Candidates should demonstrate awareness that feature selection is an iterative process that often requires experimentation and domain expertise.
8. How would you handle a dataset with a significant class imbalance?
A strong response should demonstrate understanding of the challenges posed by imbalanced datasets and various strategies to address them. The candidate might suggest:
- Resampling techniques:
- Oversampling the minority class (e.g., SMOTE)
- Undersampling the majority class (e.g., random undersampling)
- Combination methods like SMOTEENN or SMOTETomek
- Algorithm-level approaches:
- Using algorithms that handle imbalance well (e.g., decision trees)
- Adjusting class weights or thresholds
- Ensemble methods like BalancedRandomForestClassifier
- Generating synthetic data
- Collecting more data for the minority class if possible
Look for candidates who discuss the importance of choosing appropriate evaluation metrics (e.g., precision, recall, F1-score, AUC-ROC) for imbalanced datasets. They should also mention the need to understand the business impact of false positives vs. false negatives when deciding on an approach. Strong answers will emphasize that the choice of method depends on the specific problem, dataset characteristics, and business constraints.
9. How would you design and interpret an A/B test for a new feature on a website?
An effective answer should outline a structured approach to A/B testing and its interpretation. The candidate might describe the following steps:
- Define clear objectives and hypotheses for the test
- Determine the key metric(s) to measure success
- Calculate the required sample size for statistical significance
- Randomly assign users to control (A) and treatment (B) groups
- Run the test for a predetermined duration
- Analyze results using statistical methods (e.g., t-tests, chi-squared tests)
- Consider practical significance alongside statistical significance
- Account for potential confounding factors and biases
Look for candidates who emphasize the importance of setting up proper controls and avoiding common pitfalls like peeking at results too early or running too many tests simultaneously. Strong answers will also mention the need to consider long-term effects and the potential impact on different user segments. Candidates should demonstrate an understanding of the balance between statistical rigor and business practicality in interpreting test results.
10. How would you approach forecasting demand for a new product with limited historical data?
A comprehensive answer should demonstrate creativity and analytical thinking in dealing with limited data. The candidate might suggest:
- Leveraging data from similar products or markets
- Utilizing external data sources (e.g., economic indicators, industry trends)
- Applying qualitative forecasting methods like the Delphi technique or expert opinions
- Using analogous forecasting by finding comparable product launches
- Implementing time series models with limited data (e.g., simple moving averages, exponential smoothing)
- Considering Bayesian methods to incorporate prior knowledge
- Scenario analysis to account for uncertainty
Look for candidates who emphasize the importance of clearly communicating the limitations and assumptions of the forecast. Strong answers will mention the need for frequent re-evaluation and updating of the forecast as more data becomes available. Candidates should also discuss how to balance different data sources and methods to create a robust forecast, and how to present results in a way that acknowledges the inherent uncertainty.
14 Data Interpretation questions related to data analysis techniques
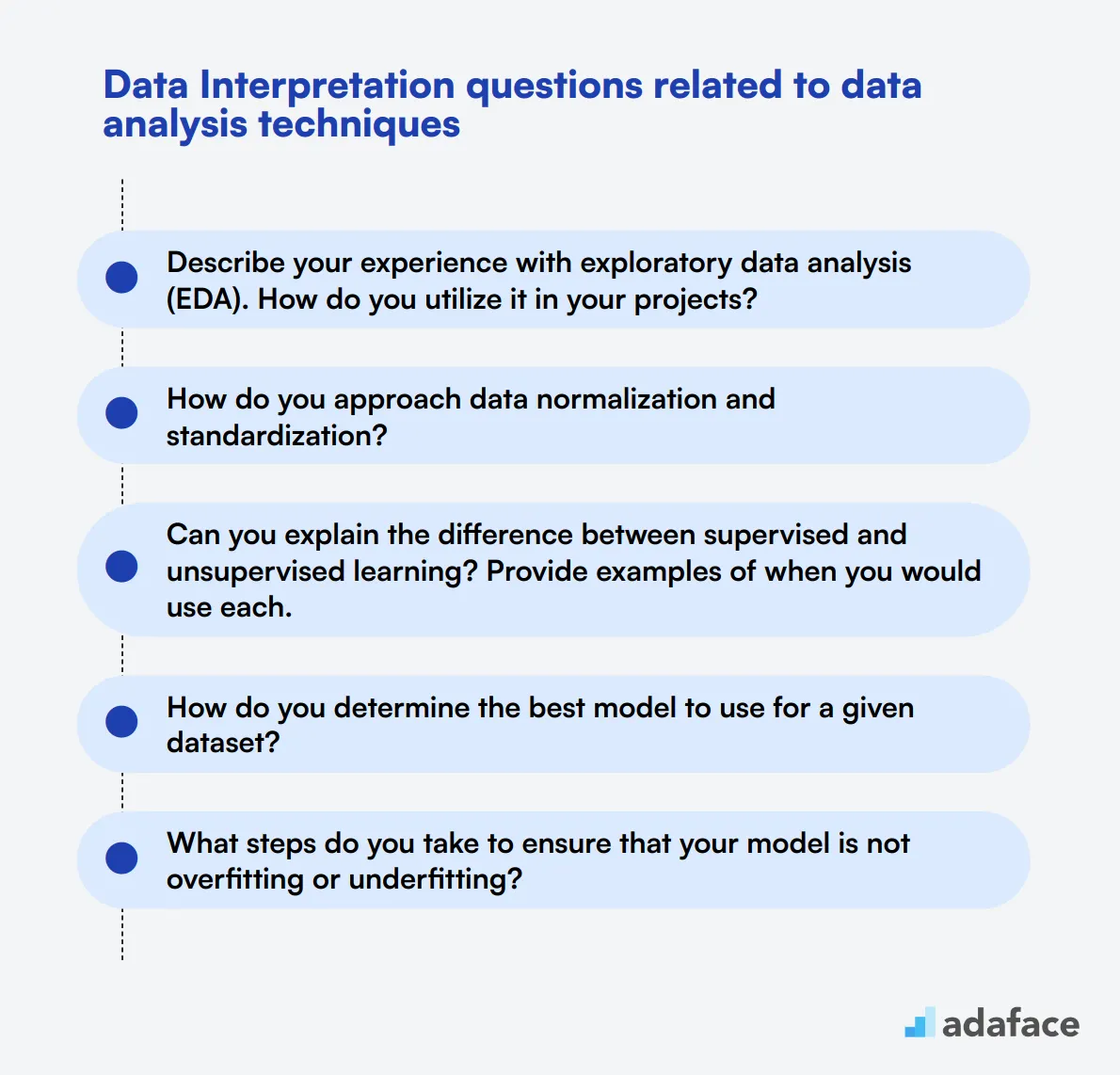
To assess a candidate's proficiency in data analysis techniques, consider these targeted questions. They will help you evaluate if the applicant possesses the necessary skills and insights to effectively analyze and interpret complex data sets, ensuring they are a great fit for your data analyst job description.
- Describe your experience with exploratory data analysis (EDA). How do you utilize it in your projects?
- How do you approach data normalization and standardization?
- Can you explain the difference between supervised and unsupervised learning? Provide examples of when you would use each.
- How do you determine the best model to use for a given dataset?
- What steps do you take to ensure that your model is not overfitting or underfitting?
- Can you discuss a time when you had to optimize a machine learning model? What techniques did you use?
- How do you handle categorical data in your analyses?
- What tools and libraries do you prefer for data manipulation and why?
- Describe your approach to performing a root-cause analysis.
- How do you utilize clustering techniques in your data analysis work?
- What is your process for feature engineering, and why is it important?
- How do you incorporate external data sources into your analysis?
- Can you explain the concept of data transformation? Provide an example of how you have applied it.
- How do you assess the quality and reliability of a data source before using it in your analysis?
8 Data Interpretation interview questions and answers related to data visualization methods
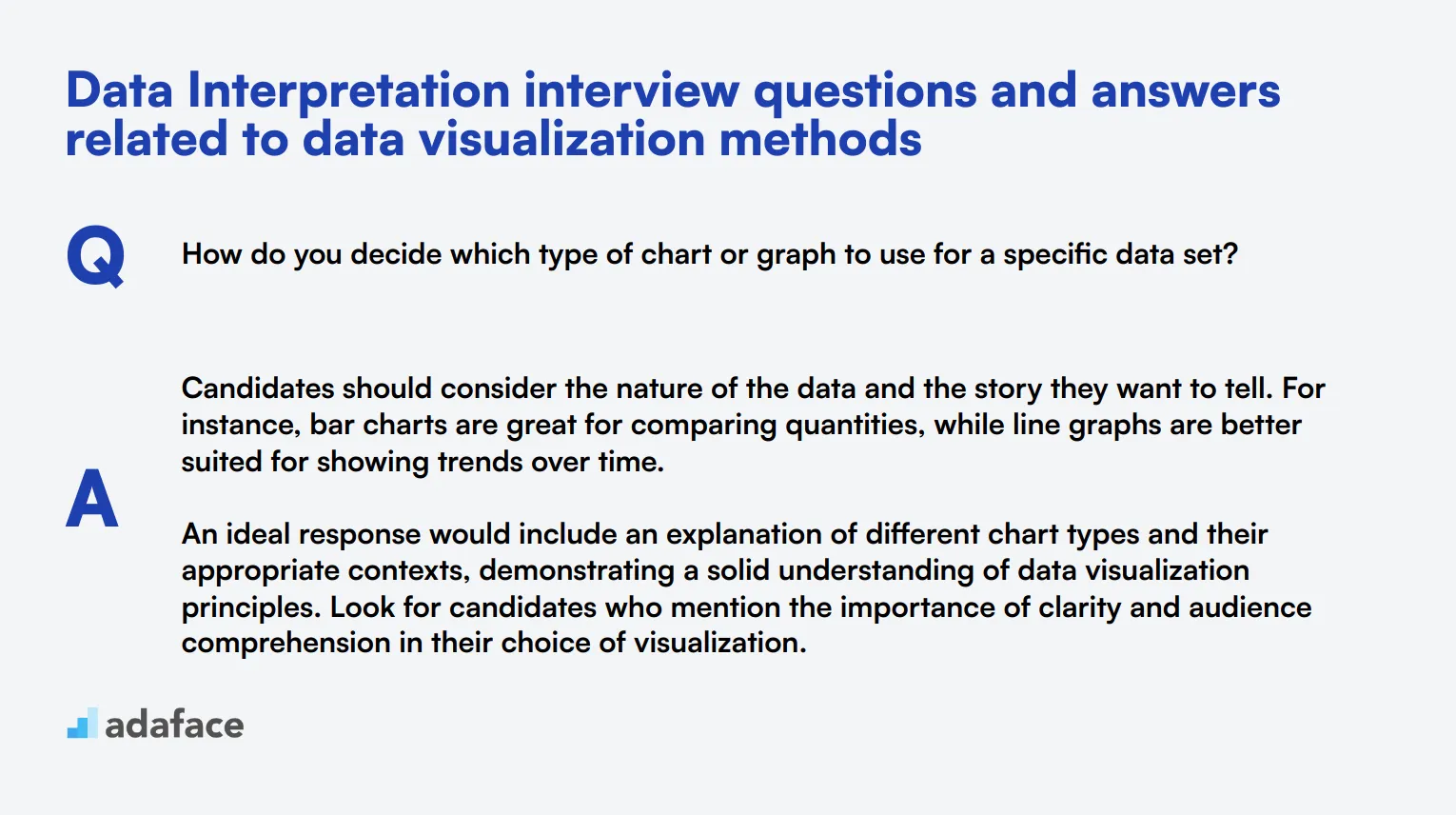
Data visualization can turn complex data sets into easily digestible insights, making it a crucial skill for any data analyst or scientist. Use these targeted questions to assess your candidates' proficiency in data visualization methods and ensure they can effectively communicate data-driven insights.
1. How do you decide which type of chart or graph to use for a specific data set?
Candidates should consider the nature of the data and the story they want to tell. For instance, bar charts are great for comparing quantities, while line graphs are better suited for showing trends over time.
An ideal response would include an explanation of different chart types and their appropriate contexts, demonstrating a solid understanding of data visualization principles. Look for candidates who mention the importance of clarity and audience comprehension in their choice of visualization.
2. Can you explain the importance of color in data visualization?
Color plays a crucial role in highlighting key data points and ensuring the visualization is easily interpreted. Candidates should talk about using color to draw attention, differentiate categories, and maintain a clear and accessible design.
A strong candidate will also mention avoiding the use of too many colors or colors that are hard to distinguish. They might refer to color theory principles or accessibility standards, ensuring the visualization is effective for a wide audience.
3. How do you ensure that your visualizations are accessible to all audiences, including those with color blindness?
Candidates should discuss using color palettes that are color-blind friendly and incorporating text labels or patterns to differentiate data points. They might also mention testing their visualizations with tools designed to simulate color blindness.
Look for responses that demonstrate a commitment to inclusivity and an understanding of the various accessibility challenges people face. The candidate should show they are proactive in ensuring their visualizations can be understood by everyone.
4. What steps do you take to avoid misrepresenting data in your visualizations?
Candidates should focus on adhering to ethical standards, such as not manipulating axes to exaggerate trends or selectively presenting data. Transparency and accuracy are key.
An ideal candidate will mention peer reviews or validation steps they take to ensure their visualizations are truthful and unbiased. They should also be aware of common pitfalls like misleading scales or cherry-picking data.
5. Can you describe a time when your data visualization skills helped solve a problem or provided a key insight?
Candidates should provide a real-life example, detailing the problem, the data visualization method they used, and the outcome. They should explain how their visualization helped stakeholders understand the data and make informed decisions.
Look for specific details and outcomes that highlight the candidate’s impact. Strong responses will demonstrate both technical skills and the ability to communicate insights effectively to non-technical audiences. More on this can be found in data analyst job descriptions.
6. How do you handle large datasets when creating visualizations?
Candidates should talk about techniques like data sampling, aggregation, or focusing on key metrics to manage large datasets. They might also mention using tools designed to handle big data efficiently.
An ideal response will include a discussion of the trade-offs involved, such as balancing detail with clarity. Candidates should show they can maintain the integrity of the data while still creating meaningful and interpretable visualizations.
7. What is your process for ensuring the accuracy and reliability of your visualizations?
Candidates should discuss their workflow, which might include data cleaning, validation checks, and peer reviews. They should also mention using reliable data sources and transparent methodologies.
Look for a systematic approach to quality assurance. Ideal candidates will demonstrate attention to detail and a commitment to producing accurate, reliable visualizations that stakeholders can trust.
8. How do you stay current with the latest trends and tools in data visualization?
Candidates might mention following industry blogs, attending webinars, or participating in professional networks. Staying up-to-date with new tools and techniques is crucial for continuous improvement.
An ideal candidate will show curiosity and a proactive approach to learning. They should be able to discuss recent trends or tools they have adopted and how these have enhanced their work.
8 situational Data Interpretation interview questions with answers for hiring top analysts
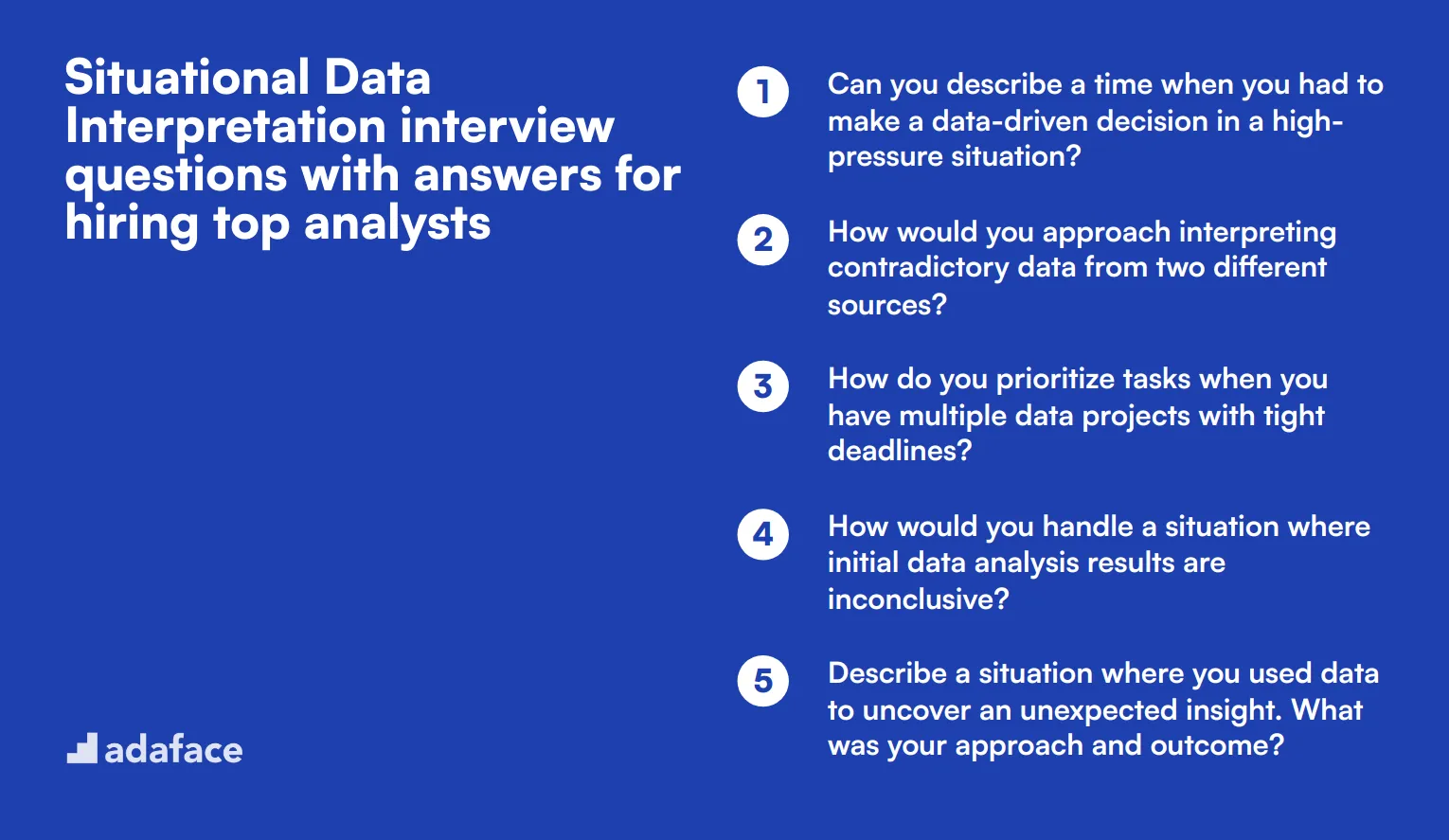
These situational data interpretation interview questions will help you assess candidates' analytical abilities and problem-solving skills. Use them during your interviews to identify top analysts who can turn data into actionable insights.
1. Can you describe a time when you had to make a data-driven decision in a high-pressure situation?
In a high-pressure situation, candidates should be able to demonstrate their ability to remain calm and methodical. They might describe using a systematic approach to evaluate data quickly, relying on their experience and tools to derive actionable insights.
A strong answer would include specific examples of the data-driven decision, the tools and techniques used, and the impact of the decision on the business outcome. Look for candidates who can clearly articulate their thought process and the steps they took to ensure accuracy under pressure.
2. How would you approach interpreting contradictory data from two different sources?
Candidates should explain that the first step is to validate the data sources to ensure their reliability. They might discuss checking the methodologies used to collect the data and considering the context in which the data was gathered.
The ideal candidate will describe steps like cross-referencing with additional data sources, consulting with stakeholders, and using statistical methods to reconcile the differences. Look for someone who emphasizes the importance of data integrity and transparency in their decision-making process.
3. How do you prioritize tasks when you have multiple data projects with tight deadlines?
Strong candidates will mention using prioritization techniques such as the Eisenhower Matrix or agile methodologies. They might explain how they assess the urgency and importance of each project and allocate resources accordingly.
An ideal response would also include examples of past experiences where they successfully managed multiple projects, outlining the steps they took to ensure timely delivery without compromising on quality. Look for evidence of effective time management and organizational skills.
4. How would you handle a situation where initial data analysis results are inconclusive?
Candidates should talk about the importance of revisiting the data collection process to ensure there are no errors or biases. They might discuss exploring alternative data sources or refining their analysis techniques to obtain more definitive results.
A strong answer would include examples of how they've dealt with inconclusive results in the past, possibly by seeking external expertise or iterating on their analysis. The key is to look for persistence and a thorough approach to problem-solving.
5. Describe a situation where you used data to uncover an unexpected insight. What was your approach and outcome?
Candidates should share a specific example where their data analysis revealed an unexpected but valuable insight. They should explain the steps they took to validate this insight and how they communicated it to stakeholders.
An ideal response would detail the impact of this insight on the business or project, demonstrating the candidate's ability to not only find hidden patterns but also to act on them effectively. Look for a strong analytical mindset and the ability to think outside the box.
6. How would you present complex data findings to a team that includes both technical and non-technical members?
Candidates should discuss their approach to tailoring their communication to the audience. They might mention using data visualization tools to simplify complex data and focusing on the key takeaways relevant to each group.
An ideal response would highlight a specific instance where they successfully communicated complex data, possibly using storytelling techniques or analogies to make the data more accessible. Look for candidates who can bridge the gap between technical and non-technical stakeholders effectively.
7. How do you ensure your data interpretations align with business goals?
Candidates should emphasize the importance of understanding the business context and objectives from the outset. They might talk about regularly consulting with stakeholders to ensure their analysis remains aligned with strategic goals.
The ideal response would include examples of how they've integrated business goals into their data analysis process in the past, ensuring their insights are actionable and relevant. Look for a strong alignment between analytical skills and business acumen.
8. Describe a time when you had to revise your analysis based on new data. How did you handle it?
Candidates should talk about their flexibility and willingness to update their findings when new data becomes available. They might describe revisiting their assumptions, re-running analyses, and communicating the changes to stakeholders.
An ideal answer would include a specific example where they successfully revised their analysis, explaining the impact of the new data and how they managed any challenges that arose. Look for adaptability and a commitment to data accuracy.
Which Data Interpretation skills should you evaluate during the interview phase?
Evaluating a candidate's data interpretation skills during an interview can be complex, as one conversation may not reveal the full depth of their capabilities. However, focusing on a few core skills can help interviewers gauge a candidate's potential effectively. Below are key skills you should consider assessing.
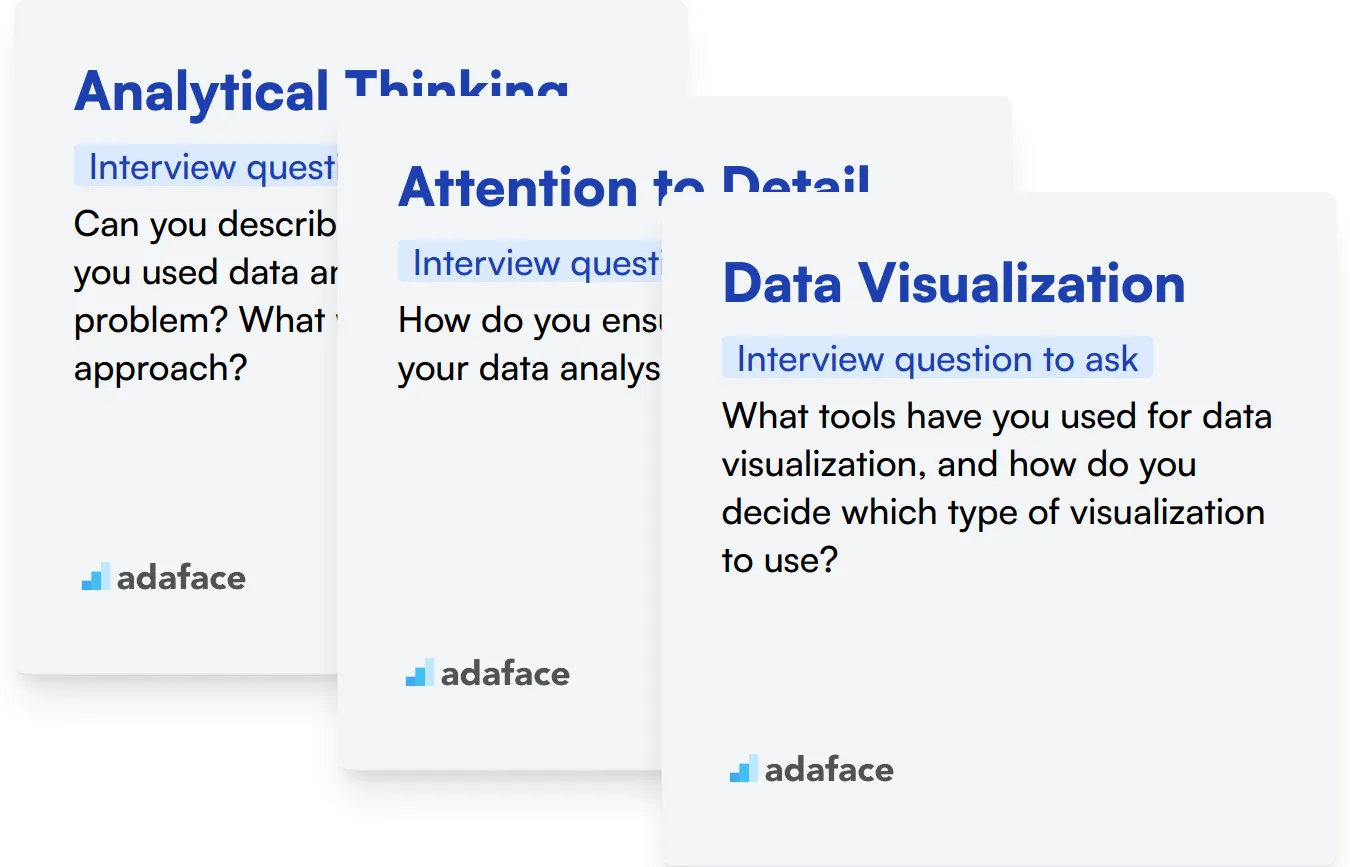
Analytical Thinking
To filter candidates based on their analytical thinking abilities, consider using an assessment test that includes relevant multiple-choice questions. Our Data Interpretation test offers a structured approach to evaluate this skill.
Additionally, you can ask targeted interview questions to further assess analytical thinking. One effective question could be:
Can you describe a time when you used data analysis to solve a problem? What was your approach?
When this question is asked, listen for specific examples that showcase the candidate's problem-solving process, tools they used, and the impact of their analysis. Look for clarity in their explanation and evidence of critical thinking.
Attention to Detail
You can gauge a candidate's attention to detail through assessment tests that include tasks requiring precision. Although we don't have a specific test for this skill, consider general quantitative reasoning assessments to filter candidates effectively.
You may also ask specific questions aimed at evaluating their detail-oriented mindset. For instance:
How do you ensure accuracy in your data analysis work?
In responses, look for mention of strategies such as thorough data checks, using software tools, and methods for self-review. Candidates should demonstrate an understanding of the importance of precision in their work.
Data Visualization
To assess candidates' data visualization skills, consider using relevant multiple-choice questions. Our Data Visualization test can serve as a resourceful tool for evaluation.
Additionally, consider asking them a practical question such as:
What tools have you used for data visualization, and how do you decide which type of visualization to use?
When asking this question, pay attention to their familiarity with various visualization tools and their ability to explain the rationale behind their choices. Good candidates should articulate how they tailor visualizations to their audience's needs.
Mastering Data Interpretation Interviews: 3 Key Tips
Before applying what you've learned, consider these tips to maximize the effectiveness of your Data Interpretation interviews. These strategies will help you assess candidates more accurately and efficiently.
1. Incorporate Skill Tests in Your Screening Process
Start by using skill tests to evaluate candidates' Data Interpretation abilities before the interview stage. This approach helps you focus on the most qualified candidates and saves time in the long run.
Consider using a Data Interpretation test to assess basic skills, or a more comprehensive Data Analysis test for broader analytical abilities. These tests can help you gauge candidates' proficiency in interpreting graphs, charts, and numerical data.
Implement these tests after initial resume screening but before interviews. This process allows you to shortlist candidates with proven skills, ensuring more productive interviews and better hiring decisions.
2. Curate a Balanced Set of Interview Questions
With limited interview time, it's crucial to select the right mix of questions that cover key aspects of Data Interpretation. Focus on questions that assess both technical skills and practical application.
Include questions that evaluate related skills such as critical thinking and problem-solving. You might also want to incorporate SQL interview questions to assess database querying skills, which are often relevant in Data Interpretation roles.
Don't forget to assess soft skills like communication and teamwork, as these are essential for effectively presenting and discussing data interpretations with stakeholders.
3. Master the Art of Follow-Up Questions
Asking thoughtful follow-up questions is key to understanding a candidate's true depth of knowledge and experience in Data Interpretation. This technique helps you distinguish between candidates who have memorized answers and those with genuine understanding and problem-solving skills.
For example, after asking about a specific data visualization technique, follow up with a question about when and why they would choose that method over others. This approach reveals the candidate's decision-making process and practical experience in Data Interpretation scenarios.
Harnessing Data Interpretation Skills to Identify Top Talent
When hiring professionals with data interpretation skills, verifying these skills accurately is key. The best way to assess these competencies is through skill tests. Consider using Adaface's Data Interpretation Test or our Data Analysis Test to ensure your candidates are well-qualified.
After using these tests to evaluate candidates, you can confidently shortlist the top performers for interviews. To take the next step in your hiring process, you can direct shortlisted candidates to sign up and further engage through our Signup Page. This streamlined approach helps you focus your interviewing efforts where they are most likely to yield the right hire.
Data Interpretation Assessment Test
Download Data Interpretation interview questions template in multiple formats
Data Interpretation Interview Questions FAQs
Focus on basic concepts, analysis techniques, visualization methods, and situational problem-solving skills.
Ask questions about various chart types, data presentation methods, and tools used for creating visualizations.
Mid-tier questions are more complex, often involving multiple data sets or advanced statistical concepts.
Use situational questions that simulate actual workplace scenarios and assess problem-solving approaches.
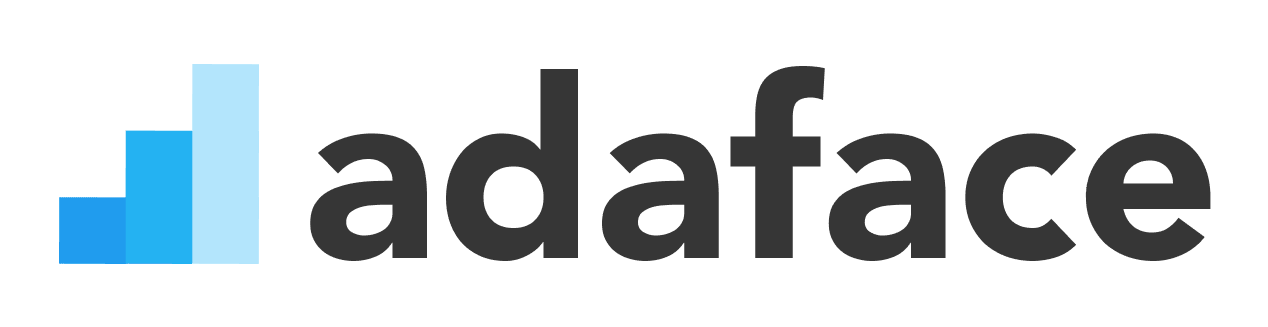
40 min skill tests.
No trick questions.
Accurate shortlisting.
We make it easy for you to find the best candidates in your pipeline with a 40 min skills test.
Try for freeRelated posts
Free resources
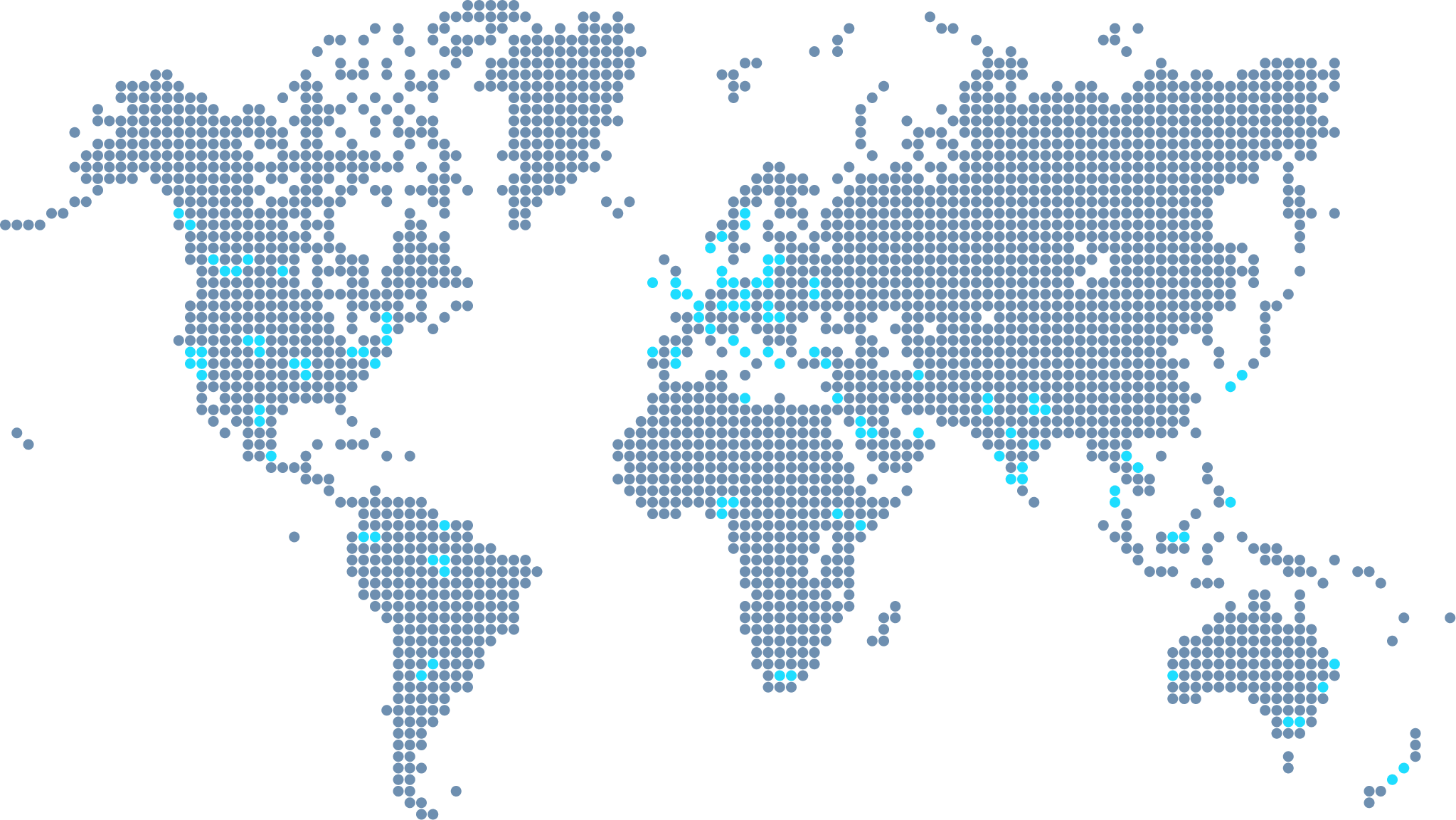
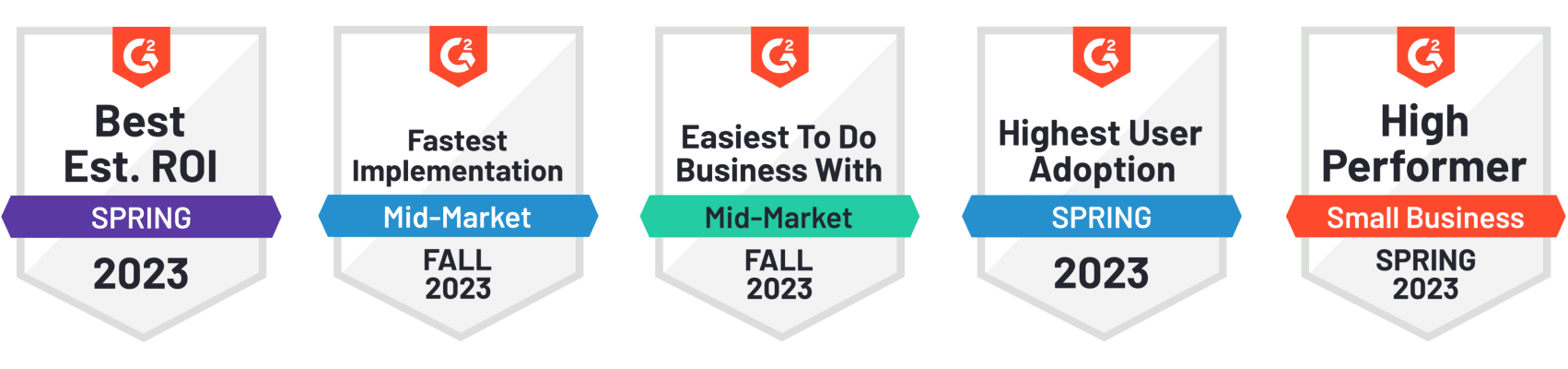